– Case Study –
Data Extraction for Mergers & Acquisitions Analytics
Leading financial intelligence company requires automation to provide hourly updates on deals
Challenge
A leading financial intelligence company offers a comprehensive database of information on M&A, IPO, private equity, and venture capital. They collect structured and unstructured data comprised of 84 fields of interest within news items from 5 sources. Because manually processing the unstructured data is both resource- and time-intensive, they sought an elegant solution for how to automate this process.
Solution
Innodata built a proprietary machine learning model trained by in-house subject matter experts that facilitates an automated approach to extracting and structuring relevant information. To ensure speed, quality, and agility this project was set up in two phases. Phase 1: Develop & train ML model with 4,000+ deal records with 20 high-frequency data points. Phase 2: Offer continuous training and automation for 500+ deal records per day. In addition to extracting 20+ relevant entities, Innodata also deployed a sophisticated NLG (natural language generation) model to rewrite headlines.
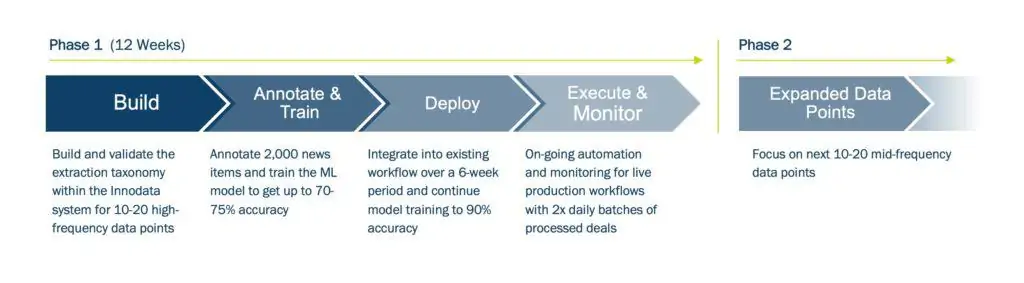
Impact
This leading financial intelligence company is able to offer hourly updates on M&A, IPO, private equity, and venture capital, making their product a world class financial resource. Innodata’s technology aids in improving turnaround time and reducing cost for deal records in the database by automating repetitive manual efforts and improving scalability across data sources. We also avoid copyright issues by rewriting headline automatically.
Meet an Expert
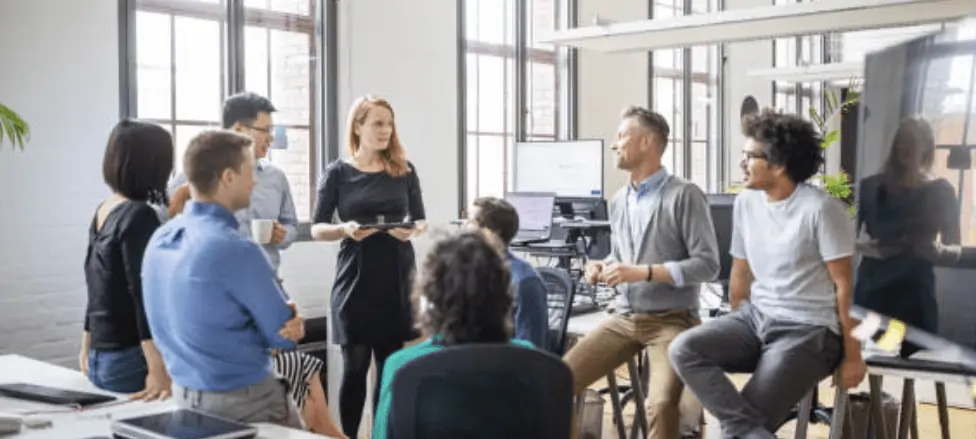
Our Team of Data Experts
A team comprised of data experts with extensive experience in developing AI-based data solutions for clients. Book a time that works for you and let us help develop a custom solution for your unique needs.