AI Data Solutions
Data Collection + Synthetic Generation
Customized Natural and Synthetic Data Collection + Creation for AI Model Training
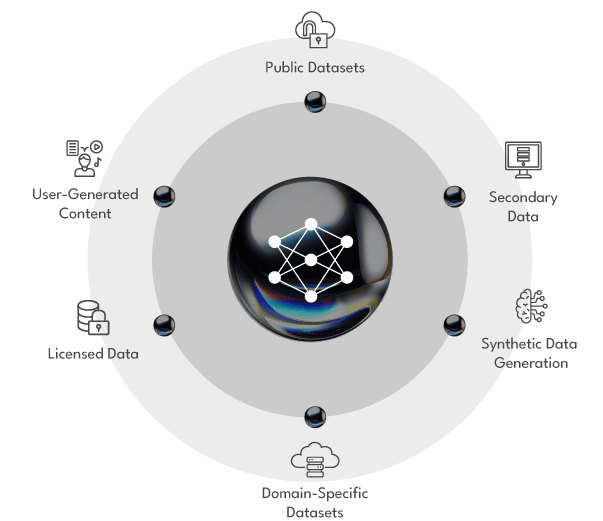
Rely on Innodata to source, collect, and generate speech, audio, image, video, sensor, text, code, and document training data for Al model development.
With 85+ languages and dialects supported across the globe, we offer customized data collection and synthetic data generation for exceptional AI model training.
Capture, Source, + Generate High-Quality Data for
Exceptional AI/ML Model Development
Innodata collects and creates customized multimodal datasets across a range of formats to help train and fine-tune AI models.
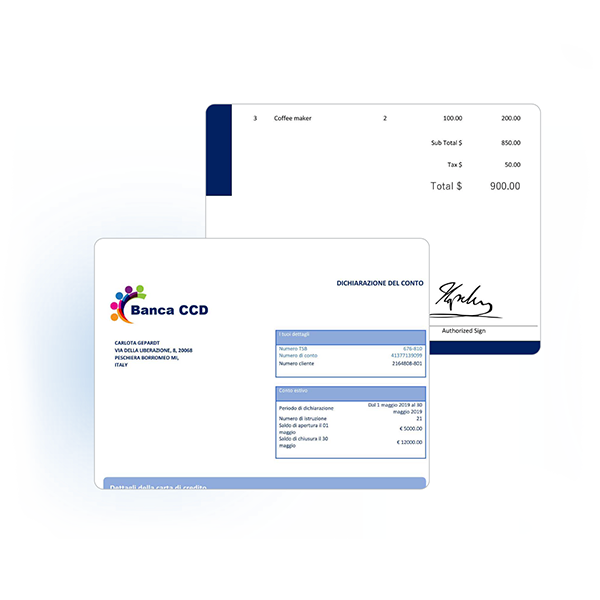
Text, Document, + Code Data
Curated and generated datasets, from prompt datasets to financial documents, and more. Scale your AI models and ensure flexibility with high-quality and diverse text data in multiple languages and formats.
Sample Datasets:
- Prompt Datasets
- Invoices
- Bank Statements
- Utility Bills
- Receipts
- Packing Lists
- And More...
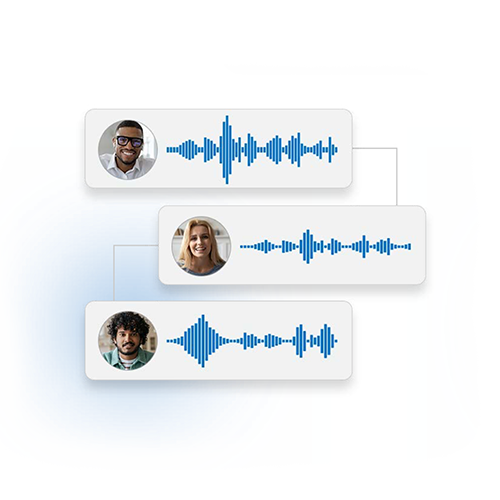
Speech + Audio
Data
Diverse datasets to train your AI in navigating the complexities of spoken language. Specify your needs from languages, dialects, emotions, demographics, to speaker traits for focused model development.
Sample Datasets:
- Customer Service Calls
- Telehealth Recordings
- Podcast Transcripts
- Lecture Recordings
- Ambient Soundscapes
- Voice Messages
- And More...
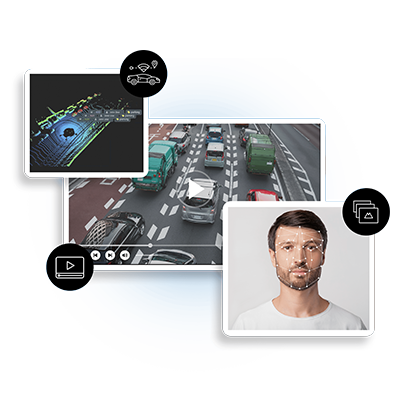
Image, Video, + Sensor Data
High-quality sourced and created data capturing the intricacies of the visual world. Empower generative and traditional AI model use cases ranging from image and video recognition to generation, and more.
Sample Datasets:
- Selfie Camera Recordings
- Retail Product Images
- Surveillance Footage
- Autonomous Vehicle Sensor Data
- Facial Data
- Sports Videos
- And More...
Synthetic Training Data.
When real-world data falls short.
Innodata goes beyond real-world data collection to offer comprehensive synthetic data creation. Synthetic data is generated data that statistically mirrors real-world data. This empowers you to:
-
Augment Real-World DataExpand existing datasets with high-quality, synthetic variations, enriching your models with diverse scenarios and edge cases.
-
Ensure Privacy ComplianceGenerate synthetic replicas of sensitive data, enabling secure and compliant model training without compromising privacy.
-
Overcome Access BarriersProduce synthetic data from restricted domains, unlocking valuable insights previously out of reach.
-
Customized Data on DemandOur teams create tailored synthetic data to your specific needs, including edge cases and rare events, for highly focused model training.
Our custom datasets are designed to reflect real-world scenarios and tailored to meet specific model needs, enabling the development of more robust and versatile AI/ML models.
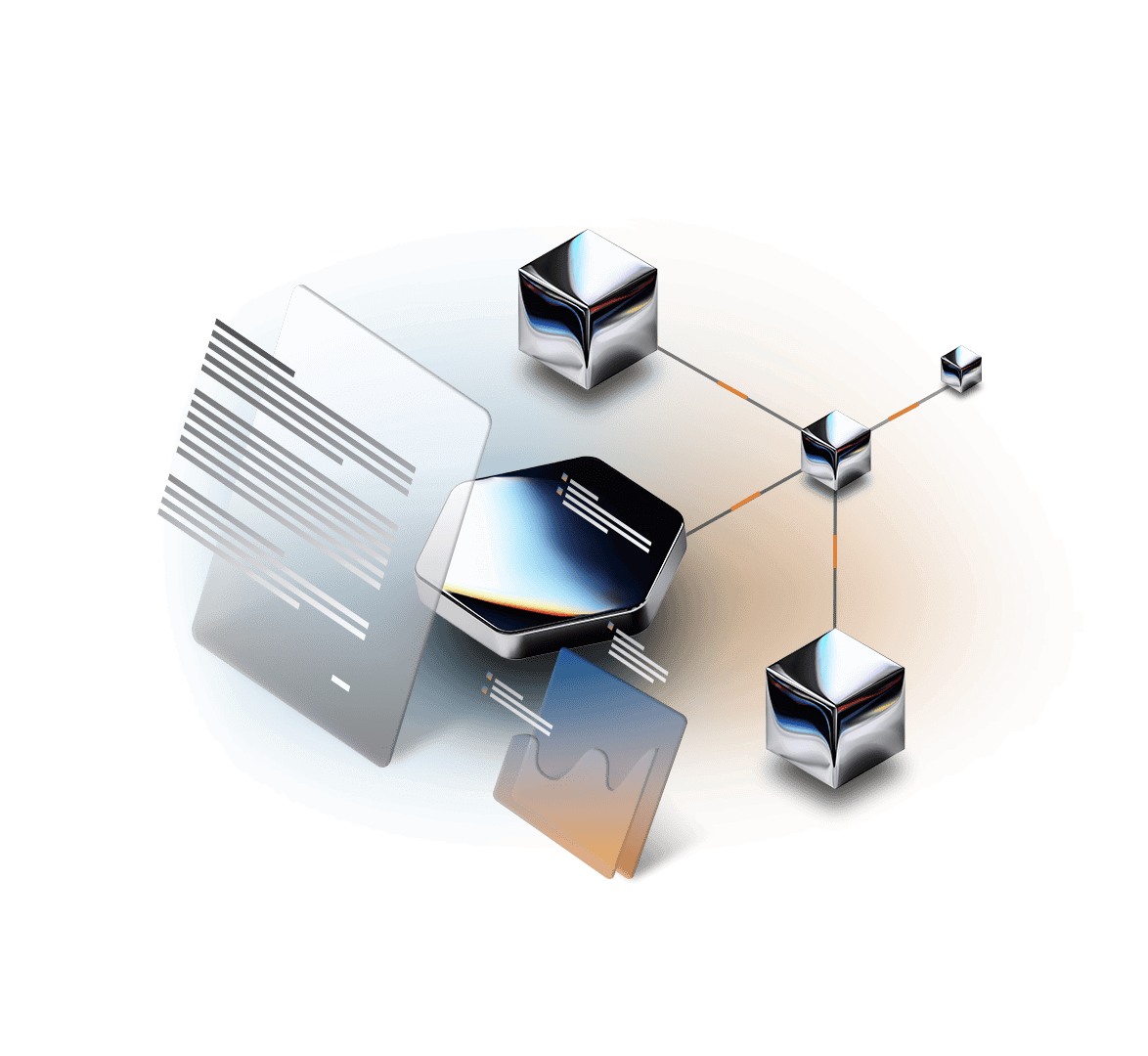
Why Choose Innodata for Data Collection + Synthetic Generation?
Bringing world-class data collection and generation services, backed by our proven history and reputation.
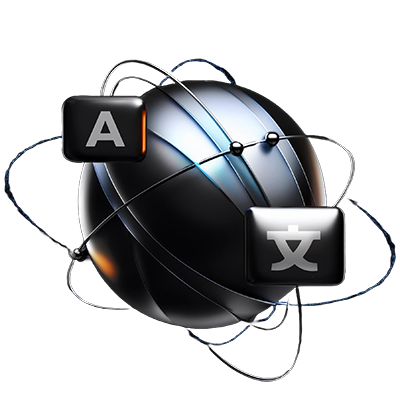
Global Delivery Locations +
Language Capabilities
85+ languages and dialects supported by 20+ global delivery locations, ensuring comprehensive language coverage for your projects.
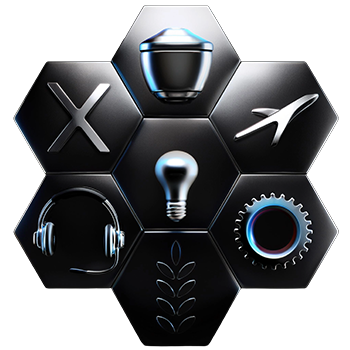
Domain Expertise Across
Industries
5,000+ in-house subject matter experts covering all major domains, from healthcare to finance to legal. Innodata offers expert domain-specific annotation, collection, fine-tuning, and more.
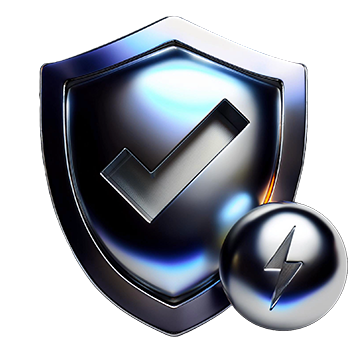
Quick Turnaround at Scale
Our globally distributed teams guarantee swift delivery of high-quality results 24/7, leveraging industry-leading data quality practices across projects of any size and complexity, regardless of time zones.
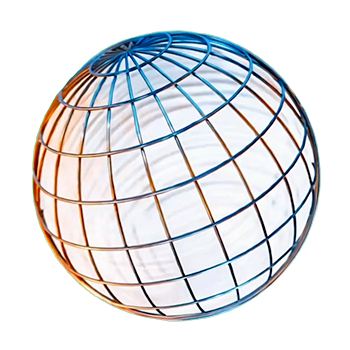
Enabling Domain-Specific
Data Collection + Creation Across Industries.
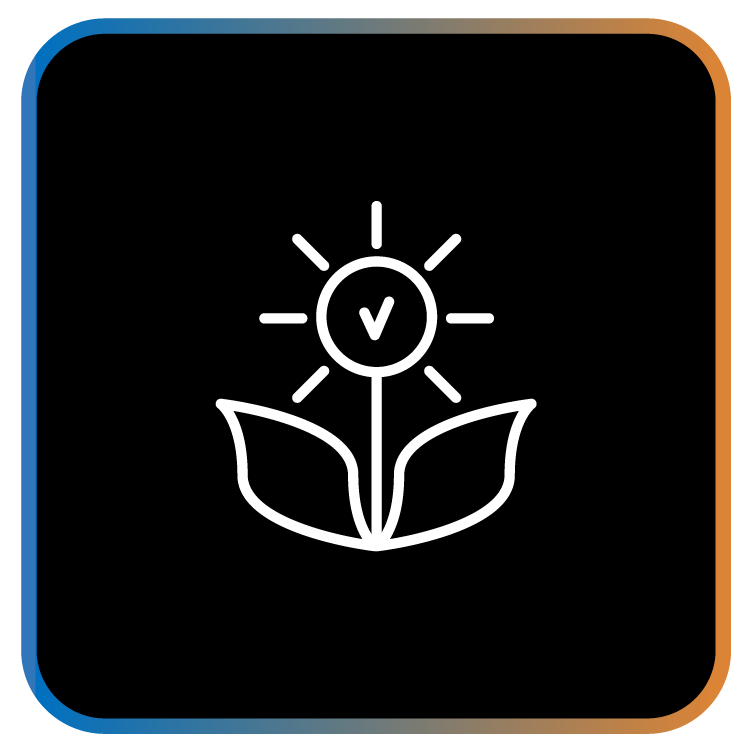
Agritech + Agriculture
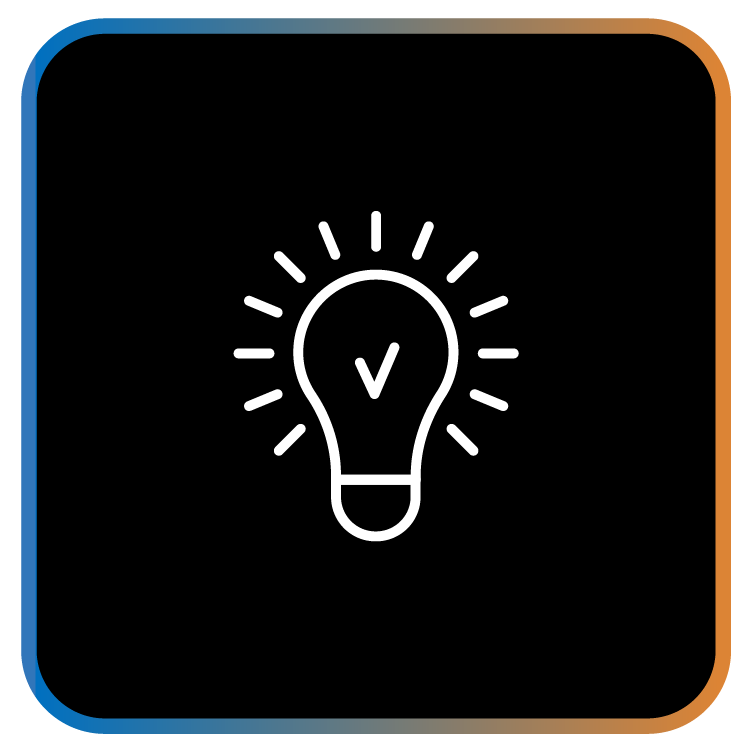
Energy, Oil, + Gas
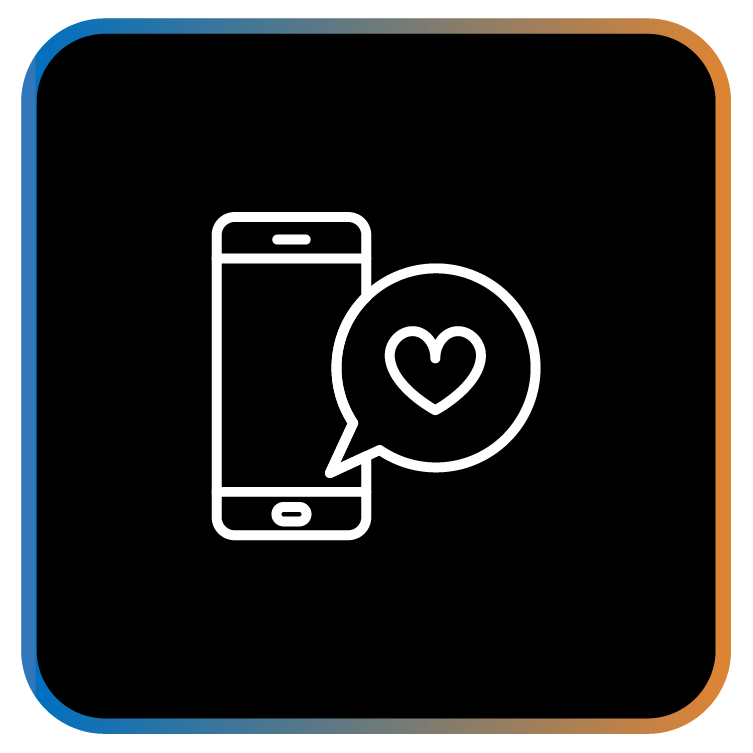
Media + Social Media
Search Relevance, Agentic AI Training, Content Moderation, Ad Placements, Facial Recognition, Podcast Tagging, Sentiment Analysis, Chatbots, and More…
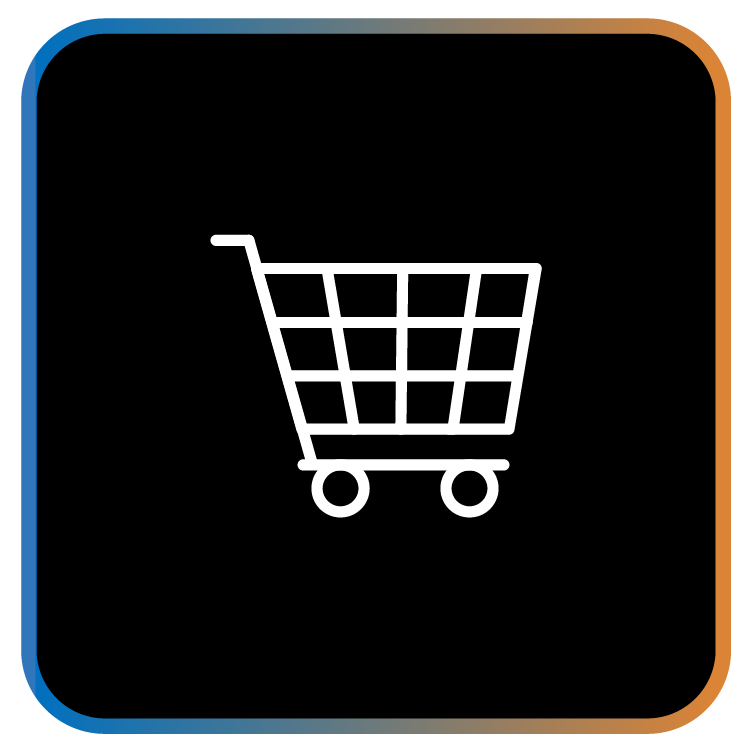
Consumer Products + Retail
Product Categorization and Classification, Agentic AI Training, Search Relevance, Inventory Management, Visual Search Engines, Customer Reviews, Customer Service Chatbots, and More…
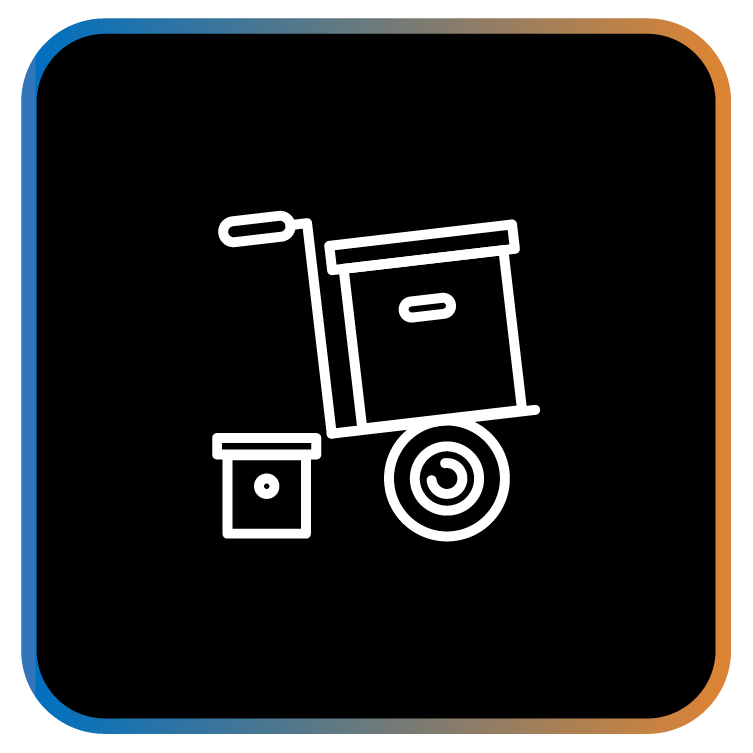
Manufacturing, Transportation, + Logistics
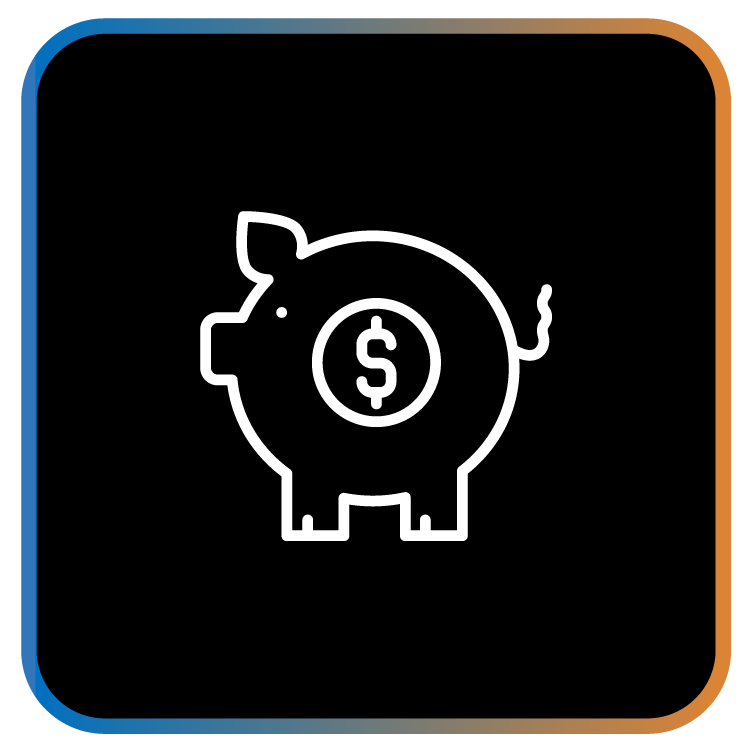
Banking, Financials, + Fintech
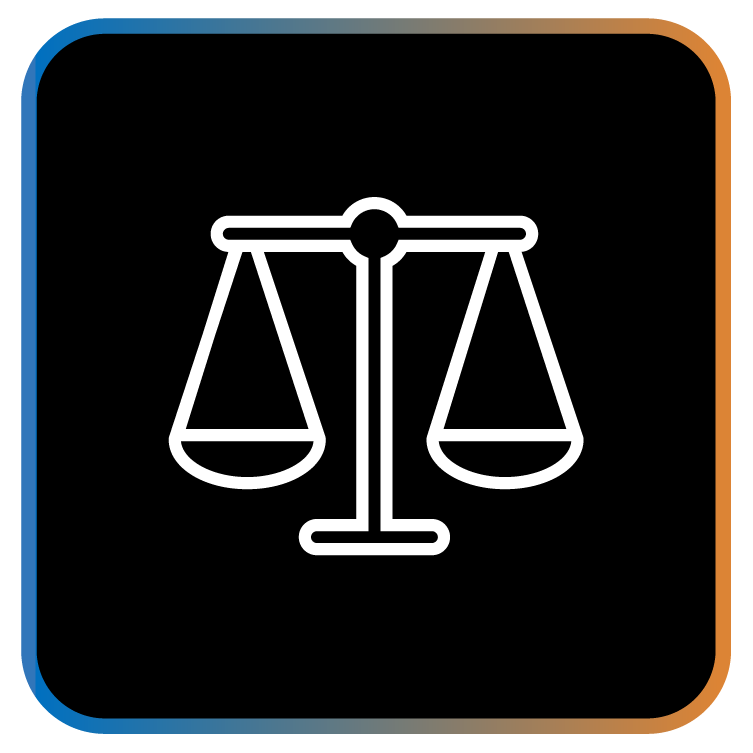
Legal + Law
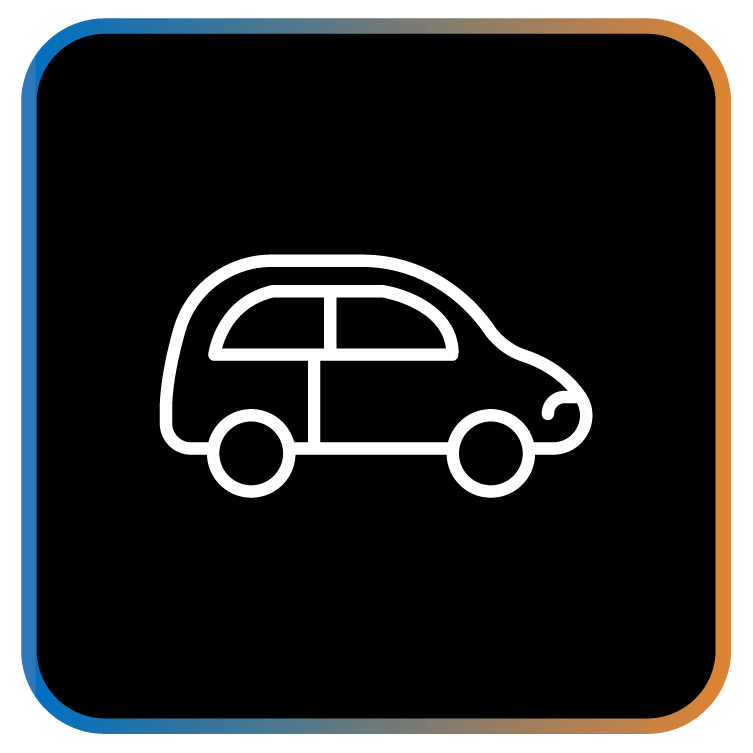
Automotive + Autonomous Vehicles
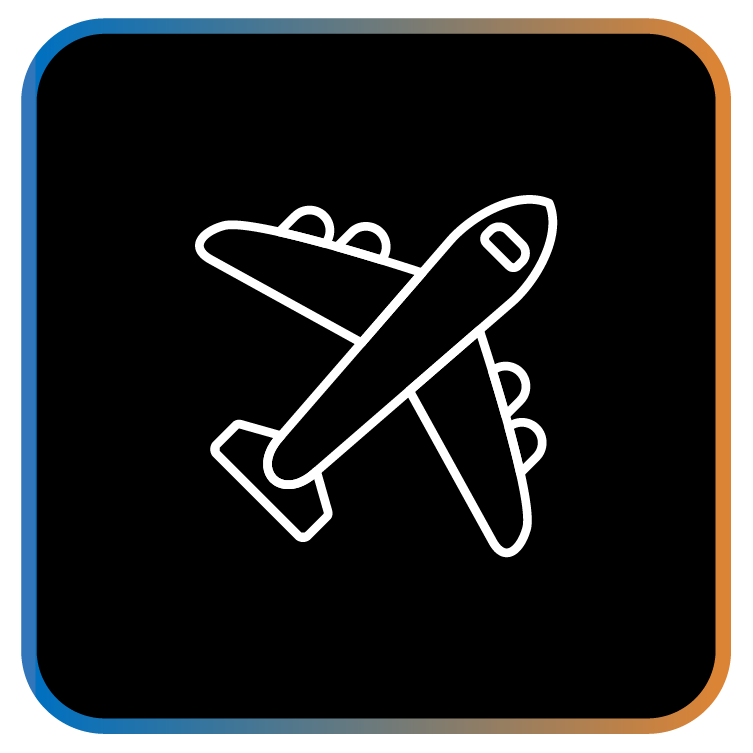
Aviation, Aerospace, + Defense
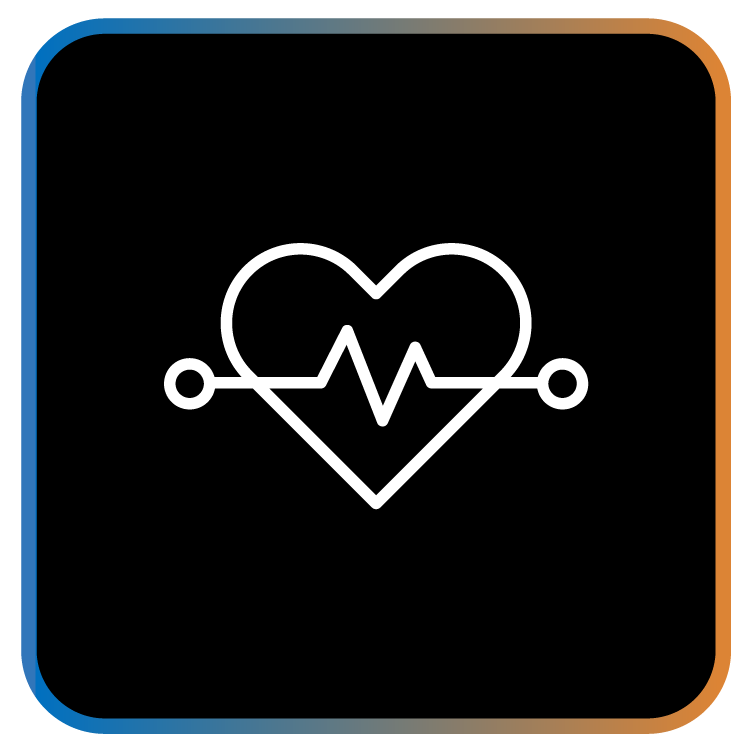
Healthcare + Pharmaceuticals
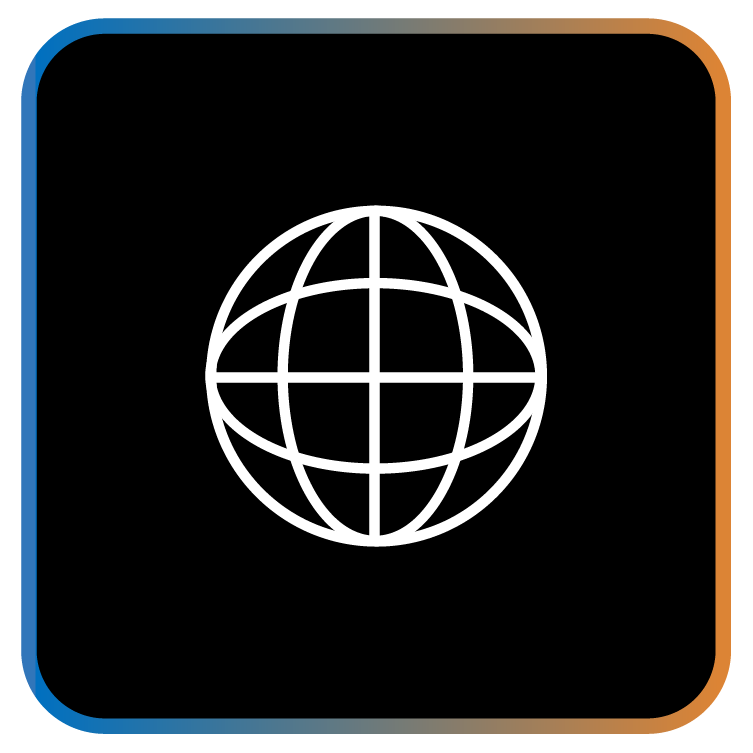
Insurance + Insurtech
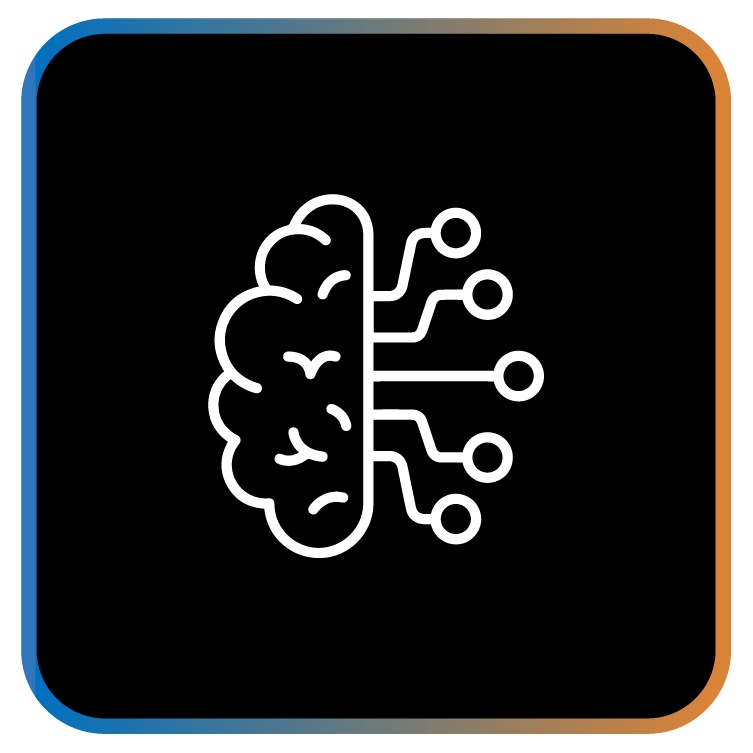
Software + Technology
Search Relevance, Agentic AI Training, Computer Vision Initiatives, Audio and Speech Recognition, LLM Model Development, Image and Object Recognition, Sentiment Analysis, Fraud Detection, and More...
Let’s Innovate Together.
See why seven of the world’s largest tech companies trust Innodata for their AI needs.
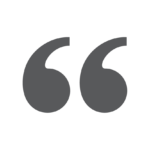
We could not have developed the scale of our classifiers without Innodata. I’m unaware of any other partner than Innodata that could have delivered with the speed, volume, accuracy, and flexibility we needed.
Magnificent Seven Program Manager,
Al Research Team
CASE STUDIES
Success Stories
See how top companies are transforming their AI initiatives with Innodata’s comprehensive solutions and platforms. Ready to be our next success story?
Data collection in AI involves gathering diverse and high-quality datasets such as image, audio, text, and sensor data. These datasets are essential for training AI and machine learning (ML) models to perform tasks like speech recognition, document processing, and image classification. Reliable AI data collection ensures robust model development and better outcomes.
Innodata provides comprehensive data collection services tailored to your AI needs, including:
- Image data collection
- Video data collection
- Speech and audio data collection
- Text and document collection
- LiDAR data collection
- Sensor data collection
- And more…
Synthetic data generation creates statistically accurate, artificial datasets that mirror real-world data. This is especially beneficial when access to real-world data is limited or sensitive. Synthetic data helps with:
- Data augmentation to expand existing datasets.
- Privacy compliance by generating non-identifiable replicas of sensitive data.
- Generative AI applications requiring unique or rare scenarios.
- And more…
Innodata offers synthetic training data tailored to your specific needs. Our solutions include:
- Synthetic text generation for NLP models.
- Synthetic data augmentation for enriching datasets with diverse scenarios.
- Custom synthetic data creation for unique edge cases or restricted domains.
- And more…
These services enable efficient AI data generation while maintaining quality and compliance.
Innodata’s data collection and synthetic data solutions support various industries, such as:
- Healthcare for medical document and speech data collection.
- Finance for document collection, including invoices and bank statements.
- Retail for image data collection, such as product images.
- Autonomous vehicles for LiDAR data collection and sensor data.
- And more…
If you’re looking at AI data collection companies, consider Innodata’s:
- Expertise in sourcing multimodal datasets, including text, speech, and sensor data.
- Global coverage with support for 85+ languages and dialects.
- Fast, scalable delivery of training data collection services for AI projects.
Yes, our synthetic data for AI solutions enhance existing datasets by creating synthetic variations. This approach supports AI data augmentation, ensuring diverse training scenarios for robust model development.
We deliver high-quality datasets, including:
- Image datasets such as surveillance footage and retail product images.
- Audio datasets like customer service calls and podcast transcripts.
- Text and document datasets for financial, legal, and multilingual applications.
- Synthetic datasets for generative AI, tailored to your specific requirements.
- And more…
Synthetic data replicates the statistical properties of real-world datasets without including identifiable information. This makes it an excellent option for training AI models while adhering to strict privacy regulations.
Data collection involves sourcing real-world datasets from various modalities like image, audio, and text, while data generation creates artificial (synthetic) data that mimics real-world data. Both approaches are crucial for building versatile and high-performing AI models.
Yes, we offer LiDAR data collection for applications in autonomous vehicles, robotics, and environmental analysis, ensuring high-quality datasets for precise model training.