How AI & ML Are Revolutionizing Supply Chain Optimization
Efficiency has always been paramount in any supply chain. The pandemic has only accentuated the need to be fully optimized and ready to adapt to sudden increases in demand or changes in the market. Businesses have turned to artificial intelligence (AI) and machine learning (ML) to plug operational gaps and improve supply chain logistics. However, implementation of such technology has plateaued in the last year, with only 12% of supply chain professionals saying that their organization employs AI in their operations.
Common challenges in supply chain optimization
The struggle to maintain unstructured data without automation
One of the main reasons for this plateau is that supply chain teams often have to manually wade through masses of unstructured data to generate insights on which to base business decisions.
However, businesses often fail to establish systems that will allow them to collect this data through software-based object recognition, image tagging, and text recognition. Even with the introduction of the Internet of Things and advanced data collection software, 62% of companies still have limited visibility of their supply chain and only 6% of companies have full visibility over their supply chain.
Market forces constantly change based on dynamic customer demand
A key challenge in logistics management is maintaining consistency in operations as customer demands change and evolve. Consistency allows supply chain executives to reliably predict key operational metrics and make strategic decisions to improve profitability and increase efficiency.
This can be difficult to do manually. Important factors such as human error and fluctuating customer demand are dynamic variables that can change significantly in short periods of time. AI can help close this gap. However, AI systems designed to generate insight often work best when built on well-defined models that follow consistent labels and rules. This can be a challenging commitment for businesses that might not have dedicated data management teams.
How intelligent software has changed supply chain operations
Fully integrated and automated systems provide holistic supply chain visibility
It can be difficult to balance the need to provide customers with virtually unlimited choices while maintaining efficiency in the supply chain. This is due to the intricacies and unique storage and delivery requirements of each product line.
Comprehensive computer vision datasets help business leaders build holistic overviews of supply chains and find patterns ripe for optimization. Amazon, in particular, has been able to utilize machine learning to identify ways to reduce the environmental impact of its product packaging. In this case, intelligent software empowered supply chain managers to find commonalities across vast product categories and delivery types.
Balancing common ground between business and customer needs
The pandemic highlighted the necessity for businesses to identify the needs of their customers and adapt to them quickly. As COVID-19 drove customers to purchase more essential products such as toilet paper and hand sanitizer, supply chain managers had to ensure sufficient inventory and efficient delivery. The same managers would have to adjust inventory levels to match decreasing customer demand in a different situation. When trained properly, AI can plug these information gaps by combining information from multiple sources and databases to build an accurate situation report that can then be translated into a supply chain strategy.
Guy Peri, chief data and analytics officer at Procter & Gamble shared with VentureBeat that “For AI to be successful, we need strong data management practitioners to ensure we have quality, reliable data going into the algorithms so the insights and actions recommended by the algorithms deliver the right outcomes for the business.”
How to leverage AI and ML to generate and analyze insightful data
Collect and organize the right types of data
Assessing the right metrics can go a long way to increasing the reliability of the insights generated by them. This means that business leaders must collect the right types of supply chain data and ensure that this data collection is standardized across the chain.
While collecting data from within the supply chain is extremely important, sometimes the data needed to train models is not available or does not exist in-house. In those cases, it can be supplemented with open-source datasets, sourced by a third-party vendor, or synthetic data can be created.
Companies that adopt AI-based solutions to collect operational data can streamline administrative processes and improve operational efficiency. 79% of executives find that AI systems simplify workflows and help them maintain high levels of efficiency across the business. In a business environment that is constantly evolving, adaptability is a critical asset.
Utilize precise annotation for model training
When training and implementing AI models to apply to supply chains, systems rely on consistent data labeling to identify items and analyze trends. Business leaders must employ precision annotation within the supply chain to remove ambiguity, particularly for subjective data.
Precision annotation allows AI and ML models to be trained using a single source of truth to mitigate bias. Even global technology powerhouses such as Microsoft and Google have recognized the importance of removing this bias and have focused recent bug bounties on AI-specific challenges. The use of precise video and image annotation early in the model training process allows supply chain executives to preemptively counter these challenges with unbiased and accurate annotation for all the items in their inventory.
Predict market trends and supply chain challenges with advanced data analysis
Predictive analytics has grown significantly in the past few years and will continue to do so in the future. A recent report revealed that 56% of supply chain operations that are not using predictive analytics currently will be doing so by 2025. Sudden market changes caused by unforeseen events and evolving business environments can cause havoc in unoptimized supply chains. Recent events such as the spread of new coronavirus variants can have a knock-on effect on customer behavior. The use of intelligent software helps business leaders identify and respond to evolving trends. This helps reduce the financial impact on the supply chain caused by wastage and operational inefficiencies.
For business leaders to maximize the benefits of using predictive analytics, there must be a strong data-driven basis for these AI-powered decisions to be made. Once the right types of data are tagged, organized, and fed to intelligent software, business leaders can predict customer demand, anticipate supply chain challenges, and react to market trends effectively.
The future for AI in supply chain operations
Ultimately, AI and ML will find their way into every part of digital supply chains. However, business leaders must put the right data foundations in place to allow these technologies to be as effective as they can be.
If you would like to find out how businesses can leverage AI and ML to fully optimize the supply chain, book a 15-minute discovery call with our Data Strategy and Innovation Team.
If you found this material helpful, please it share on social media.
Accelerate AI with Annotated Data
Check Out the Case Study on Video Annotation to Automate Global Supply Chains
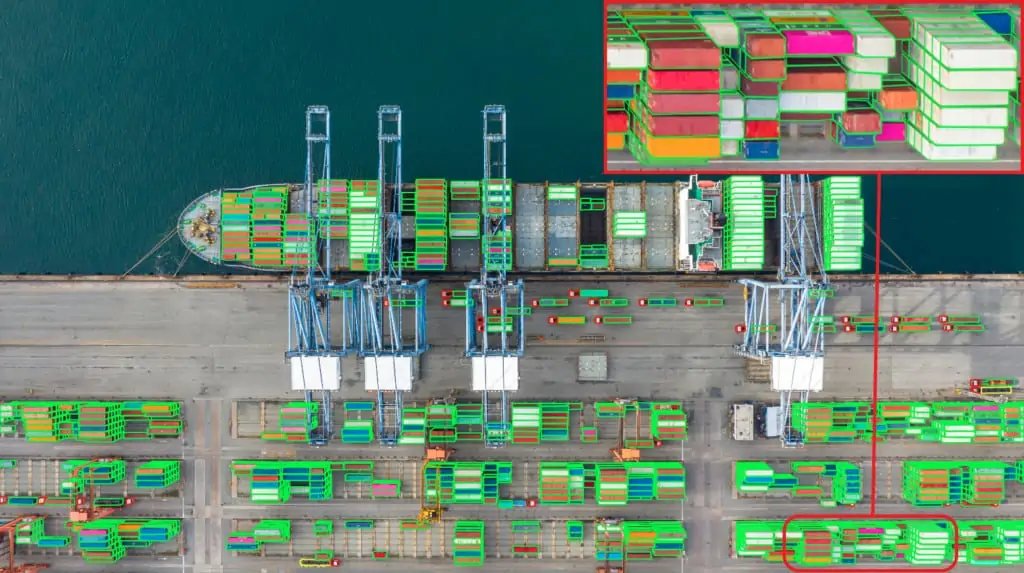