Data annotation (also referred to as data labeling) is quite critical to ensuring your AI and machine learning projects can scale. It provides that initial setup for training a machine learning model with what it needs to understand and how to discriminate against various inputs to come up with accurate outputs.
There are many different types of data annotation modalities, depending on what kind of form the data is in. It can range from image and video annotation, text categorization, semantic annotation, and content categorization.
The vast majority of problems in which AI models are being built to address them can fit into one (or many) of the below annotation tasks:
- Sequencing: text or time series from which there’s a start (left boundary) an end (right boundary) and a label. (g., recognize the name of a person in a text, identify a paragraph discussing penalties in a contract)
- Categorization: binary classes, multiple classes, one label, multi-labels, flat or hierarchic, otologic (g., categorize a book according to the BISAC ontology, categorize an image as offensive or not offensive)
- Segmentation: find paragraph splits, find an object in image, find transitions between speakers, between topics, etc. (g., spot objects and people in a picture, find the transition between topics in a news broadcast)
- Mapping: language-to-language, full text to summary, question to answer, raw data to normalized data (g., translate from French to English, normalize a date from free text to standard format)
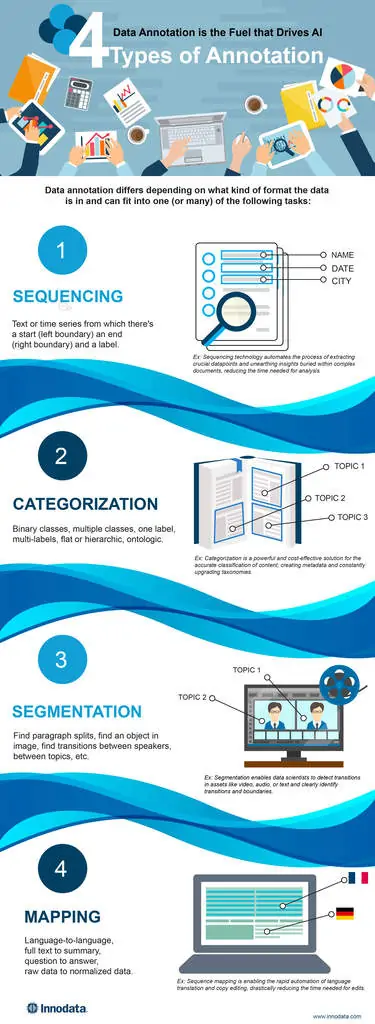
We know having access to data is quite valuable, but having access to data with a learnable ‘signal’ consistently added at a massive scale is the biggest competitive advantage nowadays. That’s the power of data annotation.