Quick Concepts
Jailbreaking Generative AI
What is Jailbreaking in Generative AI?
Jailbreaking is a form of hacking that aims to bypass an AI model’s ethical safeguards and elicit prohibited information. It uses creative prompts in plain language to trick generative AI systems into releasing information that their content filters would otherwise block.
So far, the most popular methods of jailbreaking have been to ask the AI to assume a different identity such as a fictional character or another chatbot with fewer restrictions. The subsequent prompts may include elaborate storylines or games (sometimes involving language translation, fragments of code, etc.) in which the AI is gradually coaxed into chatting about illegal acts, hateful content, or misinformation.
Purposes and Consequences of Jailbreaking
Presently, although AI model testers do conduct jailbreaks to identify and patch vulnerabilities (this is known as red-teaming), the majority of jailbreaks are conducted by users as a pastime or personal challenge. Companies such as OpenAI attempt to fight jailbreaks as they occur, but due to the volume and creativity of jailbreak efforts, this is an uphill battle.
Jailbreaking can create serious, even disastrous problems for generative AI users as the technology is adopted more widely and extensively. If everyday users are able to exploit vulnerabilities and extract safeguarded data or change a model’s behavior, cybercriminals could as easily hack into generative AI systems to access sensitive data, spread viruses, or run misinformation campaigns.
How Can Jailbreaking be Prevented or Reduced?
Worried about jailbreaking? Red Teaming can help! This proactive approach involves simulating malicious attacks on LLMs during development. Skilled data scientists, acting as ethical hackers, hunt for potential weaknesses and vulnerabilities before the LLM goes live.
This “stress test” serves two key purposes:
- Validating Defenses: By subjecting the LLM to simulated attacks, Red Teaming confirms the effectiveness of existing security measures against real-world threats. This iterative process helps refine and strengthen security protocols.
- Uncovering Blind Spots: Red Teaming challenges assumptions and pushes boundaries, uncovering vulnerabilities that might otherwise remain undetected. This proactive approach ensures continuous security improvement and mitigates potential risks.
Building an effective Red Team is not a one-time endeavor. It demands ongoing commitment and continuous adaptation. As LLM technology evolves and threat landscapes shift, Red Teams must constantly update their skills and methodologies to stay ahead of potential adversaries. This ensures the continued reliability and ethical use of LLMs in real-world applications.
For organizations venturing into the realm of LLMs, Innodata offers specialized Red Teaming services. Whether you’re building your own model or fine-tuning existing ones, Innodata’s expertise helps you implement comprehensive and effective security measures. Our tailored solutions ensure your models are resilient against potential attacks, allowing you to confidently leverage their capabilities for optimal results. Chat with a specialist today.
Bring Intelligence to Your Enterprise Processes with Generative AI
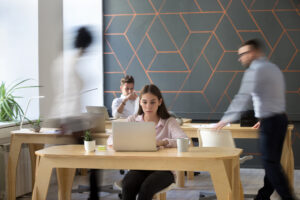
Whether you have existing generative AI models or want to integrate them into your operations, we offer a comprehensive suite of services to unlock their full potential.
follow us