Quick Concepts
What are Multimodal Large Language Models?
Artificial intelligence is rapidly evolving, mirroring human senses in groundbreaking ways. Multimodal large language models (LLMs) are at the forefront of AI, surpassing traditional text-based models by processing and understanding multiple forms of data. By combining language understanding with the ability to process images, sounds, and more, these models offer a new level of human–machine interaction. This article explores the transformative power of multimodal LLMs, delving into their core technologies, diverse applications, and the ethical implications of their development.
What Are Multimodal LLMs?
Multimodal LLMs are a new frontier in artificial intelligence capable of understanding and generating information across multiple formats, such as text, images, and audio. Unlike traditional language models that are confined to textual data, multimodal LLMs possess the ability to process and interpret the world through various sensory inputs, much like humans.
By fusing data from different modalities, these models gain a deeper comprehension of context and meaning. For instance, a multimodal LLM could analyze a social media post by not only understanding the text but also interpreting the accompanying image and background audio, providing a more nuanced response. This holistic understanding is achieved through complex algorithms and neural networks that learn to recognize patterns and relationships between different data types.
Essentially, multimodal LLMs bridge the gap between human perception and machine intelligence, enabling them to tackle a wider range of tasks and applications.
The Evolution and Importance of Multimodal LLMs
The development of multimodal LLMs addresses the inherent limitations of unimodal systems, which can struggle with tasks requiring the integration of diverse data types. Human cognition is inherently multimodal—we process visual, auditory, and textual information in a seamless blend. Mimicking this complexity, multimodal LLMs can enhance AI’s capacity to perform tasks that were previously out of reach.
Industries like healthcare, e-commerce, and robotics stand to gain significantly from these advancements. For instance, in healthcare, multimodal LLMs could enhance diagnostics by combining text-based medical records with visual data from scans and lab results, leading to more accurate and timely decisions. In e-commerce, these models could power product recommendations by analyzing both customer reviews and product images, while in robotics, they could enable machines to navigate and interact with their environments more effectively by processing visual and textual inputs simultaneously.
How Do Multimodal LLMs Work?
The Transformer Architecture
At the core of these models is the Transformer architecture. Originally developed for natural language processing, Transformers have proven remarkably effective in handling multimodal data. This architecture employs an encoder-decoder structure and an attention mechanism that allows the model to focus on relevant parts of the input. This capability is crucial for understanding the intricate relationships between text, images, and other data types.
Data Fusion: Bringing It All Together
A key challenge in multimodal LLMs is effectively combining information from different sources. Data fusion techniques bridge this gap by creating a unified representation of multimodal data. There are primarily three approaches to data fusion:
- Early Fusion: Combines data from different modalities at the very beginning of the processing pipeline. This method can be efficient but may limit the model’s ability to capture nuances within each modality.
- Mid-Fusion: Integrates data at intermediate stages of processing, allowing for a more nuanced understanding of the relationships between different data types.
- Late Fusion: Processes data from each modality independently and combines the results at the final stage. This approach can be beneficial when dealing with heterogeneous data but might miss potential synergies between modalities.
By mastering these core technologies, multimodal LLMs are able to process and generate human-like outputs across various modalities, creating exciting possibilities for applications in fields such as computer vision, natural language processing, and beyond.
Applications of Multimodal LLMs
The ability of multimodal LLMs to process and generate data across multiple modalities opens new possibilities across various industries:
- Enhanced Generative AI: Multimodal LLMs can create richer, more diverse content by integrating text with relevant images, videos, or audio. This capability is revolutionizing user experiences and enabling more sophisticated AI-driven creativity.
- Smarter Autonomous Systems: In autonomous vehicles and robotics, multimodal LLMs enhance decision-making by integrating visual, textual, and auditory data from the environment, leading to safer and more efficient operations.
- Advanced Healthcare Solutions: By analyzing a combination of text, medical images, and other health data, multimodal LLMs support more accurate diagnostics and personalized treatment plans, improving patient outcomes.
- Improved Customer Interaction: AI-powered chatbots and virtual assistants can now respond to user queries not just with text but also with images and audio, providing a more interactive and engaging support experience.
- Finance and Market Analysis: In finance, multimodal LLMs can analyze complex datasets, such as combining textual financial reports with visual charts, to generate deeper insights and more accurate market predictions.
Challenges and Considerations in Deploying Multimodal LLMs
While the potential of multimodal LLMs is immense, there are significant challenges that must be addressed:
- Computational Demands: Training and deploying these models require substantial computational resources, such as hardware and electricity, making them expensive and potentially limiting their accessibility.
- Bias and Fairness: Multimodal LLMs inherit biases present in their training data, which can lead to unfair outcomes. Ensuring diverse and representative training data is crucial to mitigate these risks.
- Complexity and Transparency: The complexity of these models can make it difficult to understand how they arrive at their decisions, raising concerns about transparency and trust.
- Ethical and Environmental Impact: The development and use of multimodal LLMs must be guided by ethical considerations, including the potential environmental impact of their computational demands.
Multimodal LLMs with Innodata
As multimodal LLMs continue to evolve, they are set to play a critical role in shaping the future of AI across diverse fields. However, realizing their full potential requires not only technological advancements but also a commitment to ethical AI practices and sustainable innovation. By navigating the challenges and harnessing the capabilities of multimodal LLMs responsibly, we can unlock new levels of productivity, creativity, and understanding in an increasingly complex world.
At Innodata, we work with leading foundational LLM companies to enhance their models. By training with high-quality data, we accelerate the development of leading models, significantly reducing the required computational power. Our expertise in generative and traditional AI has enabled these organizations to achieve greater success. Leverage our comprehensive suite of services to build robust, safe, and efficient multimodal LLMs.
Contact an Innodata expert today to learn more.
Bring Intelligence to Your Enterprise Processes with Generative AI
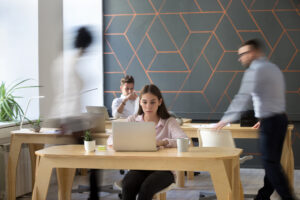
Whether you have existing generative AI models or want to integrate them into your operations, we offer a comprehensive suite of services to unlock their full potential.
follow us