Why Linguistics is Crucial for AI Model Success
In the age of AI, understanding language goes far beyond simple word recognition. AI models are expected to grasp the complexity of human communication—its nuances, tone, intent, and context. This is where linguistics steps in, acting as a guide to bridge the gap between raw data and meaningful interaction. By integrating linguistic principles into the training and fine-tuning of AI models, we can create systems that not only understand the words but also the deeper layers of meaning behind them.
The connection between linguistics and AI is more than theoretical—it’s a practical necessity. From ensuring models interpret sarcasm correctly to enabling accurate speech generation, linguistics shapes how AI models learn, process, and interact with language. As the demand for more human-like, context-aware AI systems grows, so does the need for linguistic expertise in guiding their development.
Training Data: The Foundation of AI
At the heart of every AI model lies the training data. AI models learn language patterns by processing large amounts of text from conversations, documents, and other sources. The quality, structure, and diversity of this data play a significant role in determining how well the model understands and replicates human communication. However, raw data alone is insufficient. Linguists guide the interpretation of this data by creating specific rules and frameworks that allow AI to navigate the complexities of language.
Why Linguistics is Crucial for AI Models
Linguistics provides the foundation for AI models to grasp the nuances of language. Through various linguistic branches—syntax, semantics, pragmatics, and discourse analysis—AI models can develop a deeper understanding of how humans communicate. Each branch plays a critical role in training models to interact effectively in real-world scenarios.
Syntax: Structuring Language for Understanding
Syntax refers to the rules that govern sentence structure. It ensures that words are arranged correctly to form grammatically sound sentences. AI models need to understand how different sentence components relate to each other. For instance, the difference between “The dog chased the ball” and “The ball chased the dog” is a matter of syntactic and semantic understanding. Syntax also helps models handle transformations such as questions or passive voice, allowing them to generate grammatically accurate responses in varied forms.
Semantics: Understanding Meaning Beyond Words
While syntax focuses on structure, semantics is concerned with meaning. AI models must not only recognize the literal meanings of words but also understand how meaning changes based on context. For example, the word “bank” can refer to a financial institution or the side of a river, depending on the surrounding text. A robust semantic understanding helps models disambiguate words with multiple meanings and accurately interpret figurative language like metaphors and idioms.
Pragmatics: Inferring Intent from Language
Pragmatics goes beyond the literal meaning of words to understand speaker intent. In everyday conversation, people often use indirect language, sarcasm, or polite requests. AI models trained with pragmatic principles can infer intent, adapting their responses based on context. For instance, the question “Can you pass the salt?” is a polite request, not a query about ability. Pragmatic understanding is vital for applications like customer service or virtual assistants, where interpreting user intent accurately can make the difference between helpful and unhelpful responses.
Discourse Analysis: Connecting Ideas in Extended Conversations
Discourse analysis focuses on how sentences and phrases connect within larger contexts, such as conversations or long documents. It helps AI models understand how ideas flow across multiple sentences and respond coherently in multi-turn conversations. For example, if a customer asks for help with their phone bill and later inquires, “How do I fix it?” the model must recognize that “it” refers to the phone bill. This ability is essential for maintaining consistent and relevant interactions over extended exchanges.
Navigating the Challenges of Speech Generation in AI
While text generation models have seen significant advancements, speech generation remains a complex challenge due to the additional layer of audio data requirements. Linguistic elements like phonetics and phonology are crucial in speech generation. Phonetics deals with the physical sounds of speech, such as vowels, consonants, and intonation, while phonology focuses on sound patterns within a language. By incorporating these principles, speech generation models can produce natural-sounding speech, which is essential for applications like real-time voice synthesis or virtual assistants.
Deep Learning and Linguistics: A Human Touch in AI
Large language models (LLMs) have transformed AI’s ability to process and generate human-like language, but human involvement—especially from linguists—remains crucial. While LLMs can recognize patterns from vast datasets, they lack the ability to truly understand language’s nuances, which change with context, culture, and time. Linguists ensure models evolve alongside these shifts, refining AI to capture subtleties like sarcasm, politeness, and dialects that automated systems often miss.
Linguists also play a key role in addressing bias. AI models can inadvertently reinforce societal stereotypes present in the training data. Humans help correct these biases, ensuring more balanced and inclusive datasets. Beyond bias correction, linguists advocate for representing diverse languages and dialects, making AI relevant to global audiences.
While LLMs are powerful, they lack the ethical awareness and cultural understanding that only humans can provide. As AI advances, human oversight remains essential in shaping systems that truly grasp and reflect the complexities of human communication.
Best Practices for Linguistics-Driven Model Training
To enhance AI model performance, adopting best practices in linguistics-driven training and annotation is essential. By prioritizing data quality and diversity, organizations can develop robust AI systems that effectively navigate human language complexities. Here are key strategies to optimize your AI initiatives:
- Prioritize Data Quality Over Quantity: Ensure the accuracy and consistency of labeled data to enhance model performance across languages and cultures.
- Curate Diverse Datasets: Linguists should actively reduce bias by developing and maintaining datasets that represent a wide range of languages, dialects, and cultural contexts.
- Conduct Regular Audits: Implement systematic reviews of datasets and models to identify and address biases or inaccuracies, promoting continuous improvement.
- Establish Clear, Linguistics-Informed Guidelines: Create comprehensive guidelines for annotators that incorporate linguistic principles, helping them apply consistent labeling rules and better understand ambiguous language.
- Facilitate Ongoing Training: Provide regular training sessions and feedback loops for annotators to refine their skills and understand the impact of their work on model performance.
- Encourage Collaboration with Linguists: Foster communication between annotators and linguistic experts to enhance the quality of annotations and ensure a deeper understanding of linguistic nuances.
Partnering with Innodata
As AI continues to evolve, linguistics remains essential for ensuring models not only process language but also grasp the deeper context, intent, and nuances behind it. This requires more than just advanced technology—it demands expert human guidance.
At Innodata, we leverage over 35 years of data expertise alongside linguistic experts to provide comprehensive solutions that enhance AI model performance across languages, domains, and modalities. Supporting over 85 languages and dialects and specializing in fields like healthcare, finance, law, and STEM, our expert linguists ensure your AI initiatives are culturally attuned, unbiased, multilingual, and capable of handling complex language tasks such as summarization, Q&A, and entity extraction.
Whether fine-tuning models or expanding capabilities, Innodata offers the trusted partnership, speed, and quality needed to power your AI initiatives. Connect with an Innodata expert to learn more.
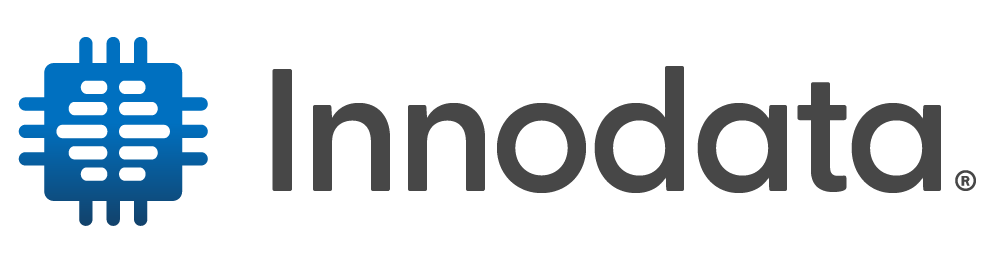
Bring Intelligence to Your Enterprise Processes with Generative AI.
Innodata provides high-quality data solutions for developing industry-leading generative AI models, including diverse golden datasets, fine-tuning data, human preference optimization, red teaming, model safety, and evaluation.
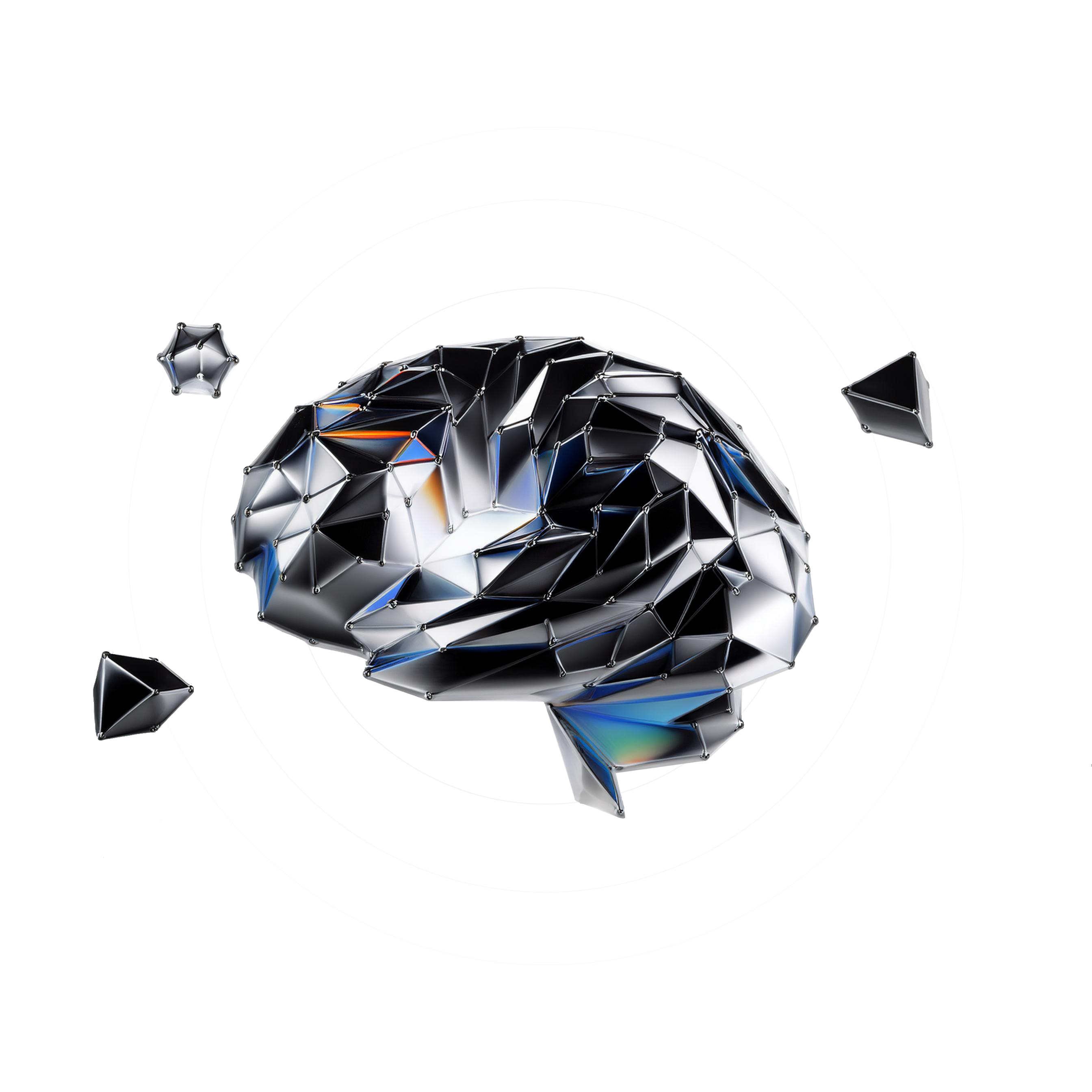
Follow Us