How to Use Multimodal AI to Drive Smarter Business Decisions: 10 Best Practices
Every day, enterprises generate and collect data in many forms—text from customer reviews, images from social media, videos from product demos, and audio from support calls. But how do you turn all of these different signals into clear, actionable decisions?
Multimodal AI enables organizations to integrate diverse data sources, uncover deeper patterns, and drive more informed business strategies. In this article, we’ll explore what multimodal AI is and share 10 best practices for using it to transform enterprise decision-making.
Understanding Multimodal AI for Decision Making
Enterprises need reliable insights that help them improve their operations. However, single-modality systems usually handle only one data type. Multimodal AI addresses this issue by integrating multiple data modalities such as text, images, audio, and videos. By combining these diverse data sources, multimodal AI provides richer insights and more accurate decision-making capabilities.
For instance, consider a fully functioning robot working in a factory. The robot must navigate, handle objects, and interact with its environment based on its commands. The operation may appear simple from the outside. However, to successfully perform these tasks, the robot needs to process visual feed, audio input, and physical action. Traditional decision-making systems could delay the robot’s responses or limit its ability to perform simple actions. However, multimodal AI systems integrate multiple data streams and enable the robot to process each command to execute tasks quickly, smoothly, and efficiently.
The Benefits of Multimodal AI in Enterprise Decision-Making
- Comprehensive Insights: Combining multiple data sources often creates a fuller picture for strategic decisions. In healthcare, numerous records of a single patient exist, including records, images from scans, and test reports. Multimodal AI can analyze these different forms of patient data to generate customized treatment plans and provide effective care.
- Real-Time Decision Making: Processing various data types simultaneously enables enterprises to make real-time decisions. Multimodal AI helps autonomous vehicles process data from multiple sensors to generate trajectories to ensure safety. These paths help driverless vehicles to successfully navigate and avoid obstacles.
- Innovation and Efficiency: Integrating multimodal AI enables enterprises to prioritize innovation and operational efficiency across multiple channels. Customer service thrives on personalized care and empathy. However, customer feedback comes through various media, including phone calls, chat support, reviews, and emails. Multimodal AI can deliver customized and seamless support through all the channels, improving the overall customer experience.
10 Best Practices for Leveraging Multimodal AI
1. Align AI Strategy with Business Objectives
Ensure your multimodal AI initiatives are integrated with your enterprise’s strategic goals. For instance, a large retail chain could use multimodal AI to combine sales data, social media feedback, and customer interactions. The integrated data allows the enterprise to fine-tune its supply chain and marketing strategies, reducing operational costs and enhancing customer engagement. Businesses can set clear, measurable outcomes, gain tangible value, and foster long-term innovation with multimodal AI integration.
2. Integrating All Diverse Data Sources Effectively
Merge structured and unstructured data to fully extract actionable insights. The information in structured formats combined with the insights hidden in unstructured data could help enterprises identify trends and patterns. For instance, a company’s financial report (structured data) might show a dip in profits, while customer reviews (unstructured data) could reveal customer dissatisfaction due to a recent product issue. This combined view of an issue allows businesses to develop targeted strategies and help them make informed decisions.
3. Data Governance
Robust data governance is essential for any AI initiative. A large-scale enterprise must manage different data formats from various touchpoints like social media, support calls, and product demos.
In this setting, governance means enforcing stringent data quality standards to ensure the integrated data is accurate, secure, and compliant with regulations. The organization can build trust in its multimodal AI outputs by regularly auditing and implementing automated quality checks tailored to each data modality.
4. Invest in Scalable Infrastructure
Multimodal AI applications require flexible IT systems that efficiently process large volumes of varied data. Investing in scalable cloud infrastructure and modern data platforms ensures that your organization can handle growing data needs without compromising performance. Scalable systems enable seamless integration and rapid adaptation, supporting the evolving demands of enterprise AI solutions.
5. Emphasize Explainability and Transparency
Multimodal AI processes diverse data types, complicating understanding its decision-making process. As AI becomes a critical part of decision-making, it’s essential that the models used can clearly explain their reasoning. Explainability builds trust among stakeholders by allowing them to understand how decisions are made. Transparent AI processes also make troubleshooting and refinement easier, ensuring that decisions are both reliable and accountable.
6. Cross-Functional Collaboration
As multimodal AI typically integrates data from various, collaboration among IT, data science, and business teams is needed to ensure cohesive, practical solutions. By creating an environment of teamwork and open communication, organizations can ensure that AI solutions are developed and deployed in alignment with practical business needs. This collaboration not only improves integration but also encourages innovation by blending diverse expertise.
7. Implement Continuous Monitoring and Model Updates
The dynamic nature and the volume of data in multimodal systems means that AI models can quickly become outdated. Continuous monitoring allows organizations to assess performance in real-time, while regular updates help models adapt to new data and emerging threats. This proactive approach ensures that AI systems remain effective and secure, continuously delivering accurate insights.
8. Address Ethical Considerations and Bias Mitigation
Ethical AI practices are critical in preventing unintended bias and ensuring fairness. Multimodal systems can inherit biases from each data type they process. Organizations must implement processes to detect and mitigate biases in data and model outputs, thereby ensuring that AI-driven decisions are just, transparent, and free from discrimination. This not only builds trust but also aligns AI initiatives with broader societal and regulatory expectations.
9. Leverage Advanced Analytics and Visualization Tools
To make sense of complex, multimodal data, it is essential to employ cutting-edge analytics and visualization tools. These tools help translate raw data into clear, actionable insights through intuitive dashboards and real-time visual representations. Such visualizations enable decision-makers to quickly grasp trends and patterns, supporting faster and more informed decisions.
10. Partner with Experts
Collaborating with industry leaders and leveraging specialized services can accelerate your multimodal AI initiatives. Partnering with experts provides access to cutting-edge technologies, best practices, and invaluable guidance that can streamline the implementation process. Tailored AI solutions and expert services can help you integrate multimodal AI seamlessly into your business, ensuring you stay ahead in the competitive landscape.
Next Steps for Smarter Decision-Making
Leveraging multimodal AI is essential for enterprises aiming to unlock deeper insights and make intelligent, data-driven decisions. By aligning AI initiatives with strategic goals, integrating diverse data sources, establishing robust data governance, and partnering with experts, organizations can gain a significant competitive edge.
Are your decision-making processes ready for the AI revolution? Connect with Innodata’s experts today to explore tailored AI solutions that empower your enterprise with smarter, faster, and more informed decision-making.
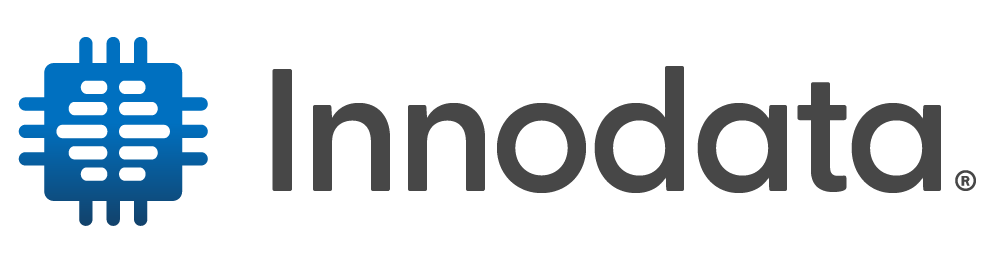
Bring Intelligence to Your Enterprise Processes with Generative AI.
Innodata provides high-quality data solutions for developing industry-leading generative AI models, including diverse golden datasets, fine-tuning data, human preference optimization, red teaming, model safety, and evaluation.
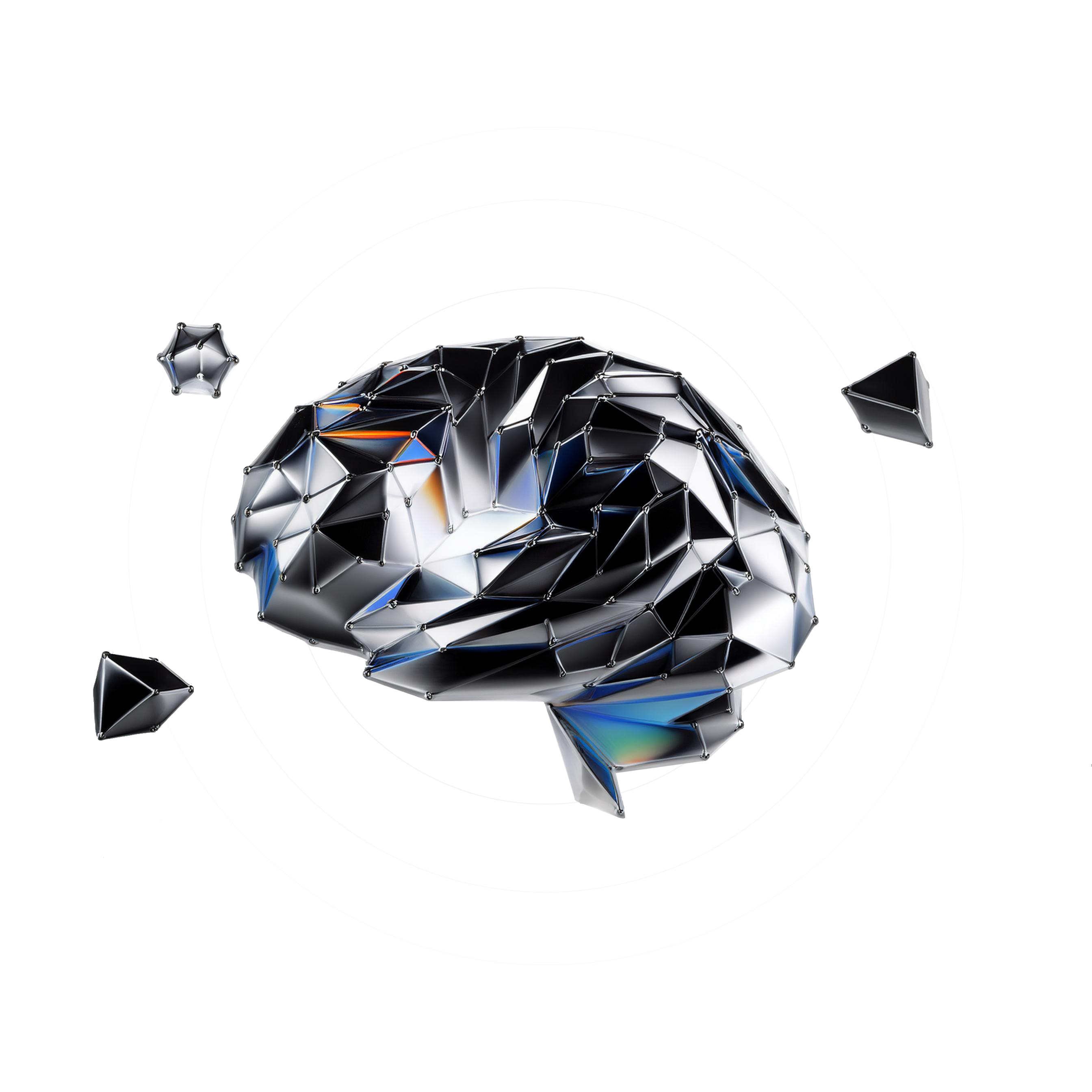
Follow Us