Generative AI Data Solutions
Implementation Services
Implementation Services
Ideate > Pilot > Implement > Maintain Implement Generative AI Into Your Enterprise
Ideate > Pilot > Implement > Maintain
Implement Generative AI Into Your Enterprise
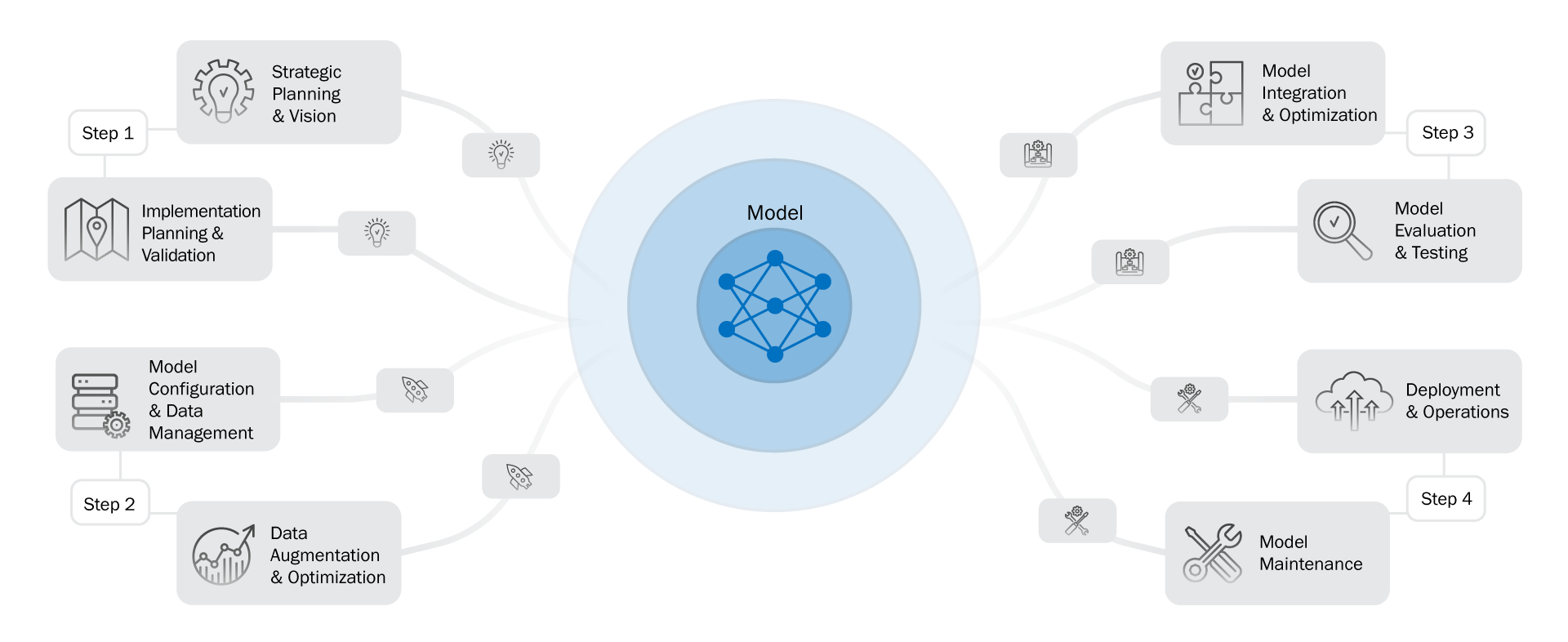
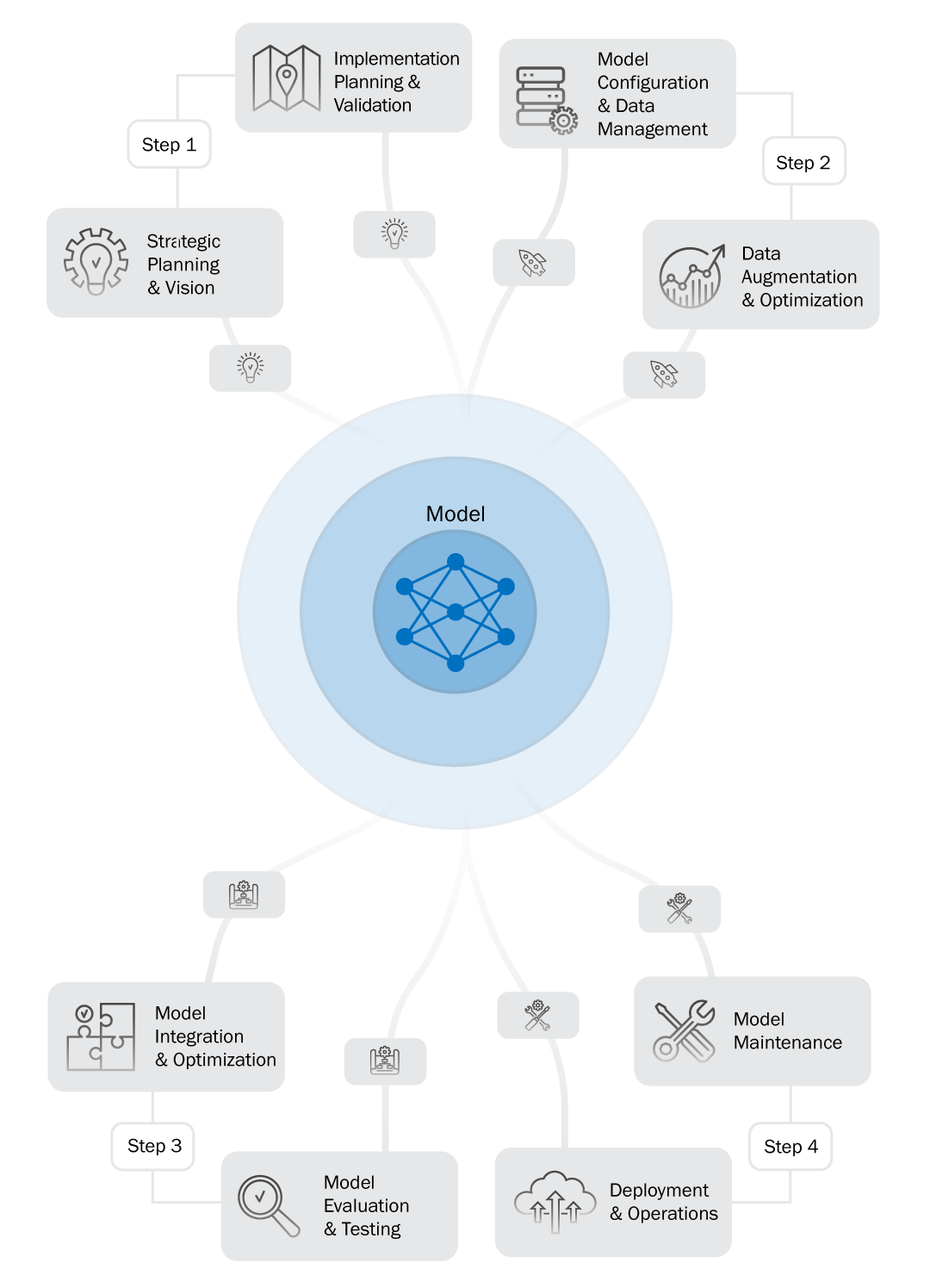
Innodata offers expertise in implementing generative AI models into enterprise business operations. From ideation to maintenance, we guide your journey through innovative vision workshops, exploratory pilot execution, seamless implementation, and ongoing model maintenance.
Innodata offers expertise in implementing generative AI models into enterprise business operations. From ideation to maintenance, we guide your journey through innovative vision workshops, exploratory pilot execution, seamless implementation, and ongoing model maintenance.
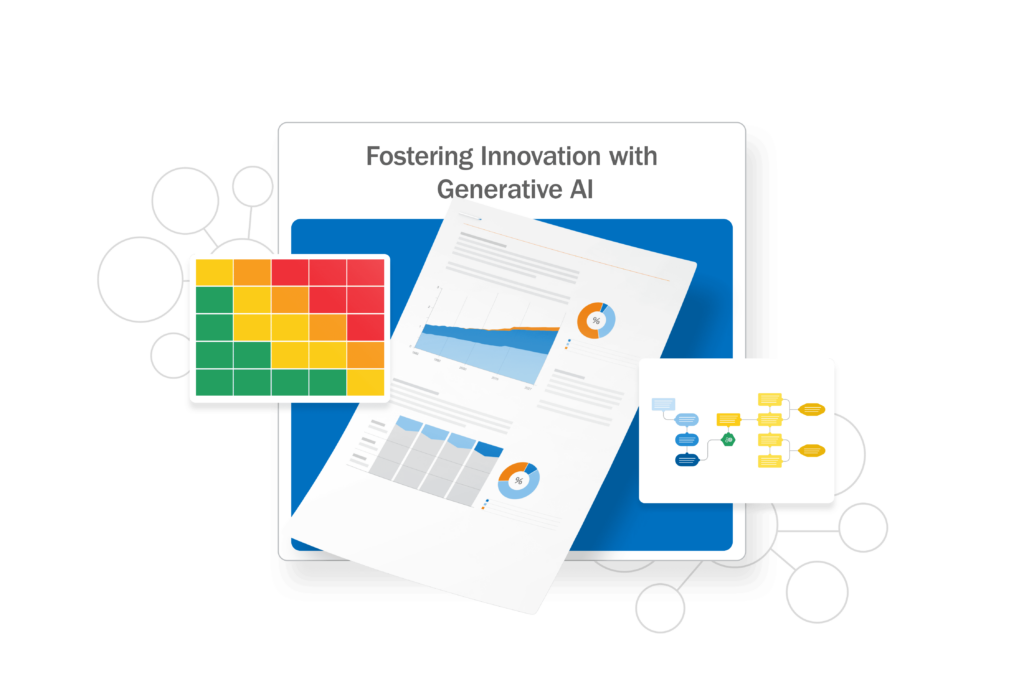
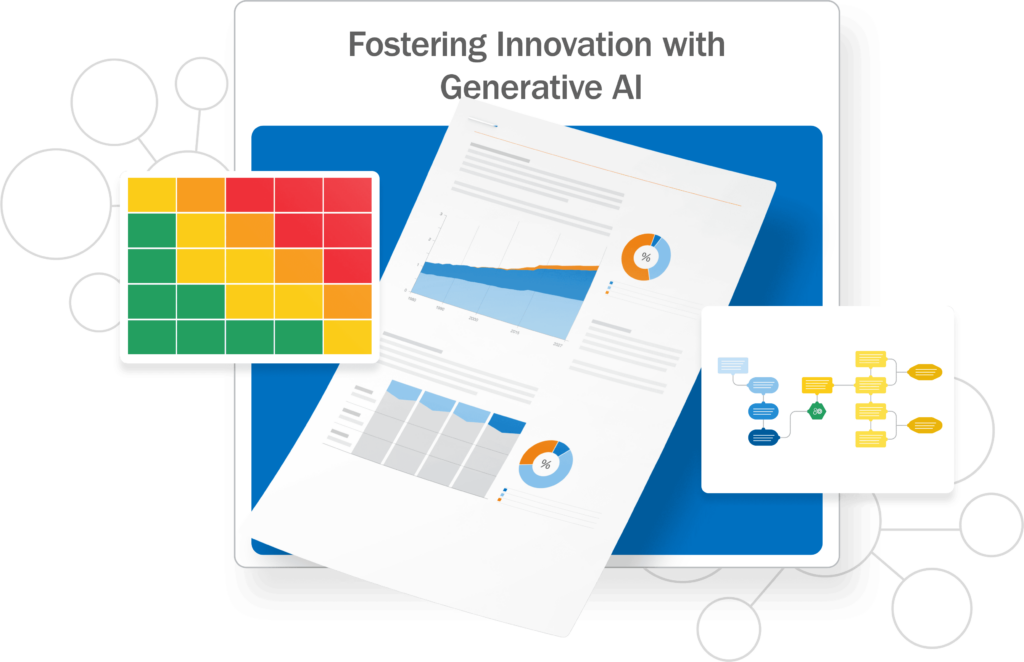
1 Ideate
1
Align with your business objectives, define automation roadmaps, and find where generative AI can foster innovation within your organization with Innodata’s consultative vision workshops. We bring design thinking and strategic planning to establish the right balance of person/machine synergy.
We Offer
Employing human-centered design principles to develop creative solutions tailored to your specific needs.
Creating a strategic roadmap outlining the steps involved in implementing your chosen generative AI solution.
Working with you to define problems, potential solutions, and expected ROI.
Analyzing your workflows to ensure integration with generative AI is feasible and beneficial.
-
Vision Workshops: Facilitating collaborative sessions to understand your business goals and challenges and identify potential areas for generative AI applications.
-
Design Thinking: Employing human-centered design principles to develop creative solutions tailored to your specific needs.
-
Roadmap Defining: Creating a strategic roadmap outlining the
steps involved in implementing your chosen generative AI solution.
-
Business Case Definition: Working with you to define problems,
potential solutions, and expected ROI.
-
Process Validation: Analyzing your workflows to ensure
integration with generative AI is feasible and beneficial.
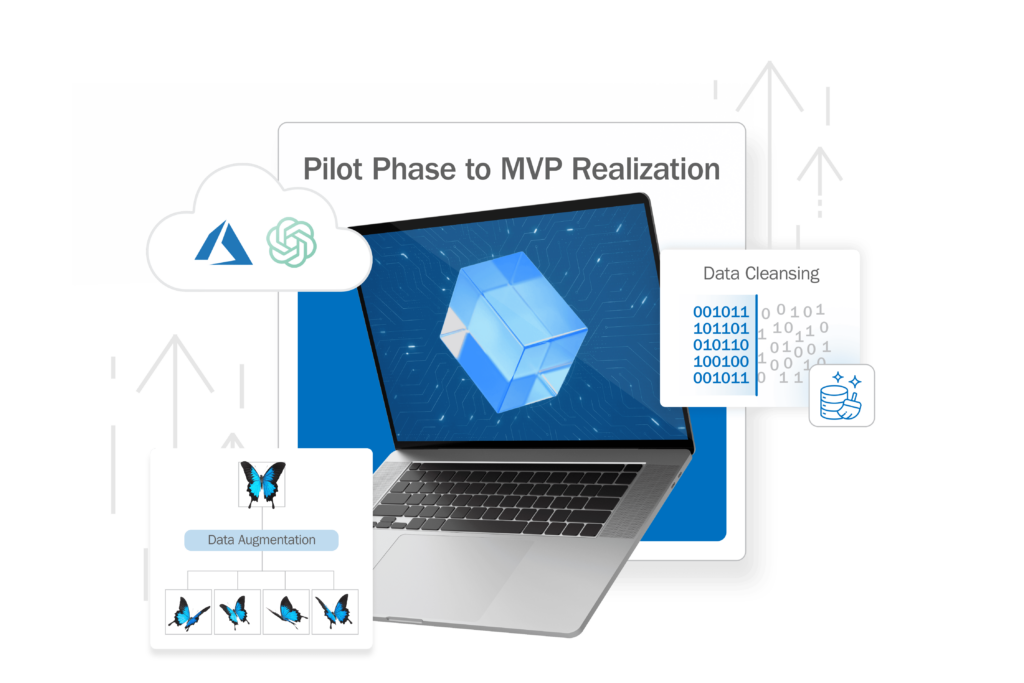
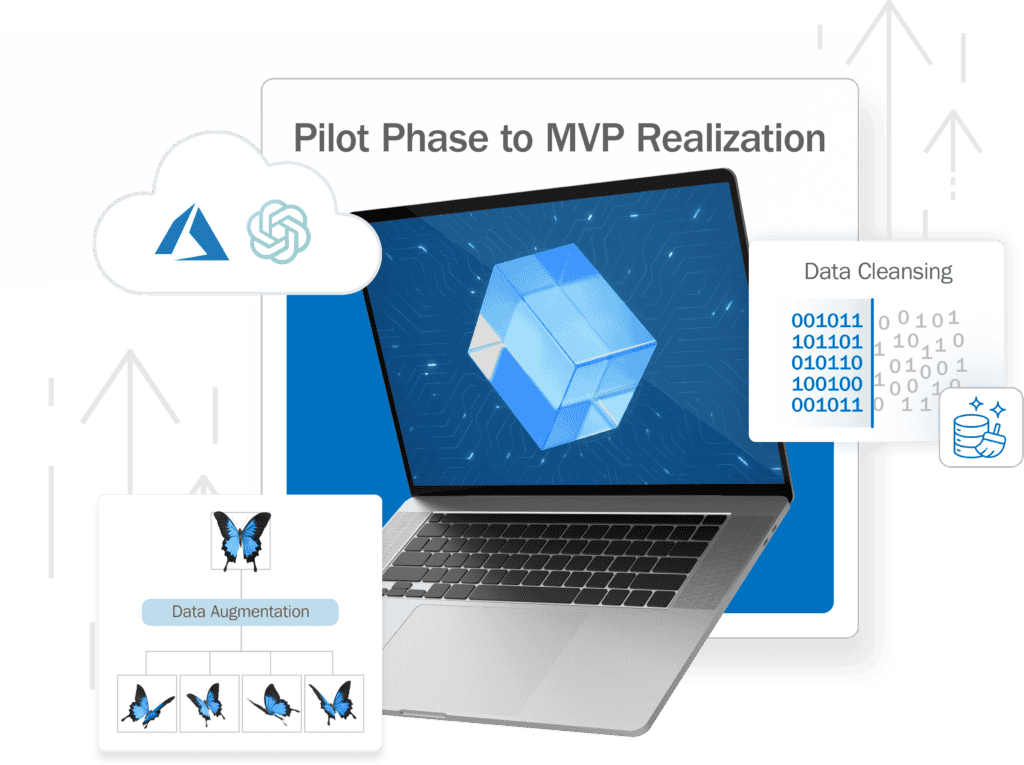
2 Pilot
2
The Pilot phase focuses on MVP (minimum viable product) realization through model configuration and data preparation for your specific business case(s). We assess and cleanse your existing data or augment it through natural data collection or synthetic generation per your specifications. If applicable, our team will also provide human preference optimization services or create data for supervised fine-tuning / fine-tune. This stage sets the foundation for full-scale implementation post-pilot.
We Offer
Our expert team will assess key model questions, such as choosing between OpenAI API or Azure Cloud, determining parameter size (e.g., 7 billion vs. 70 billion), deciding between cloud or private hosting, etc.
Our team collects your data, then carefully cleanses, and normalizes it, ensuring high-quality and readiness for model training.
According to your specifications, our teams can naturally curate or synthetically generate data over a wide range of data types and 85+ languages to address training
data limitations.
-
MVP Model Configuration: Our expert team will assess key model questions, such as choosing between OpenAI API or Azure Cloud, determining parameter size (e.g., 7 billion vs. 70 billion), deciding between cloud or private hosting, etc.
-
Data Management: Our team collects your data, then carefully cleanses, and normalizes it, ensuring high-quality and readiness for model training.
-
Data Augmentation: According to your specifications, our teams can naturally curate or synthetically generate data over a wide range of data types and 85+ languages to address training data limitations.
-
HPO and Data for Fine-Tuning: By utilizing HPO (human preference optimization) or by creating data for supervised fine-tuning, we support tuning MVP models to adapt to your specific use case(s).
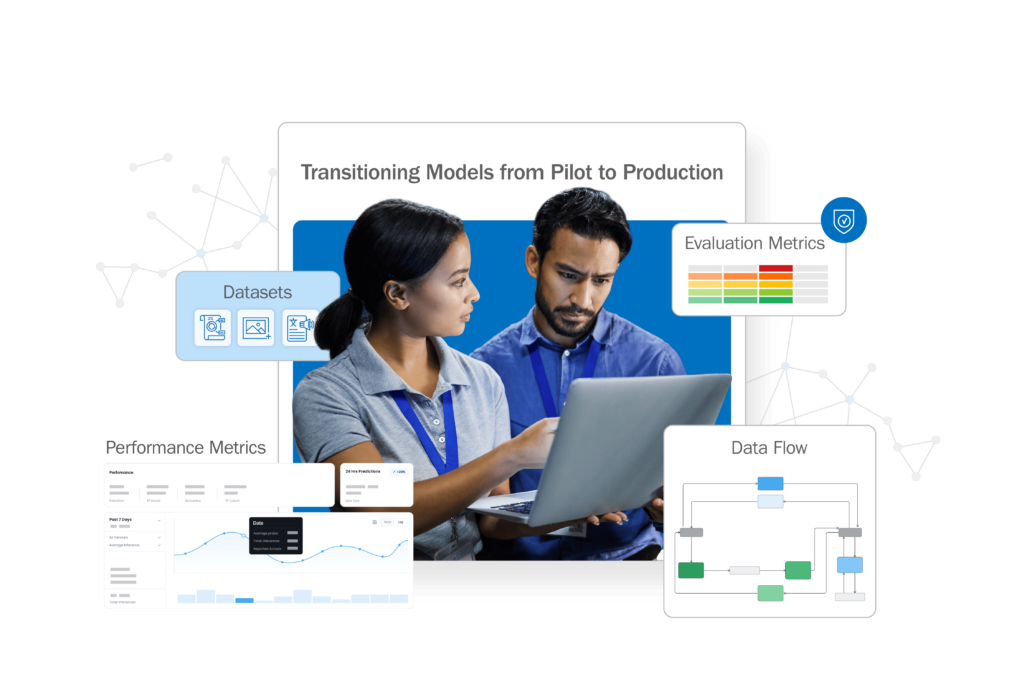
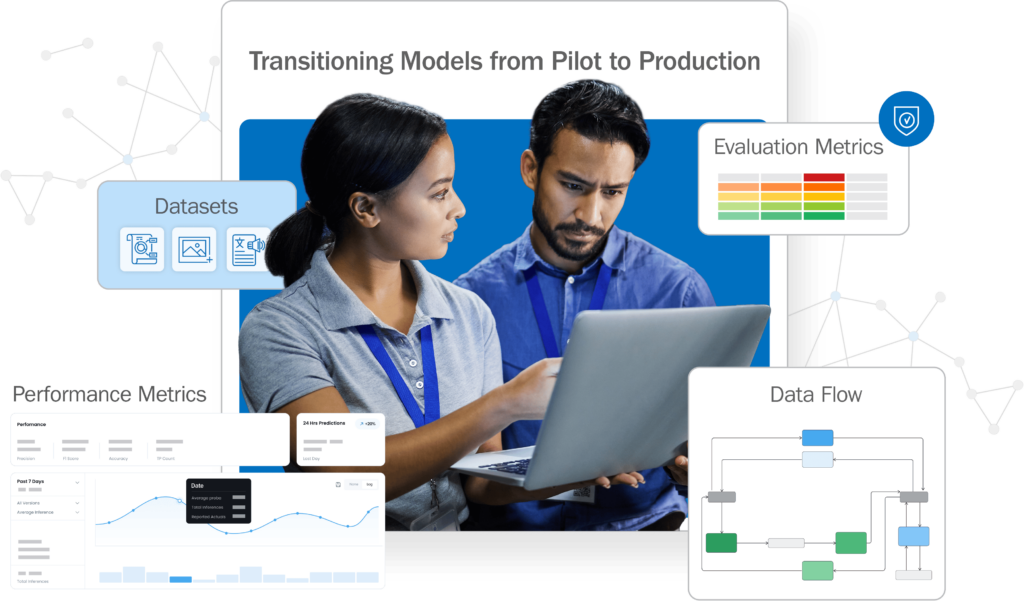
3
3 Implement
The Pilot phase focuses on MVP (minimum viable product) realization through model configuration and data preparation for your specific business case(s). We assess and cleanse your existing data or augment it through natural data collection or synthetic generation per your specifications. If applicable, our team will also provide human preference optimization services or create data for supervised fine-tuning / fine-tune. This stage sets the foundation for full-scale implementation post-pilot.
Transitioning your models from pilot to production. First, your designated implementation team will focus on integrating models into your workflows. Next, we will assist in guiding the MVP (minimum viable product) model to meet the accepted criteria for production success — utilizing techniques like natural or synthetic data collection, human preference optimization, and data creation for supervised fine-tuning. If applicable, the team will then address model evaluation, safety, and perform red teaming to address model weaknesses. And finally, user acceptance testing is conducted to ensure a successful transition to production.
We Offer
Our team seamlessly integrates your model into your existing production flows, ensuring smooth operations and efficient data flow.
According to your specifications, our teams can enrich your production training data with naturally curated or synthetically generated data over a wide range of data types and 85+ languages.
By utilizing HPO (human preference optimization) or by creating data for supervised fine-tuning, we support tuning production models for increased performance.
Red teaming experts assess model safety and weaknesses using task-specific metrics to gauge accuracy and identify potential improvements, then allowing for improved accuracy with new data.
Thorough user acceptance testing to ensure the model meets your specific requirements and delivers the expected business value.
-
Model Integration: Our team seamlessly integrates your model into your existing production flows, ensuring smooth operations and efficient data flow.
-
Data Augmentation: According to your specification, our teams can enrich your production training data with naturally curated or synthetically generated data over a wide range of data types and 85+ languages.
-
HPO and Data for Fine-Tuning: By utilizing HPO (human preference optimization) or by creating data for supervised fine-tuning, we support tuning production models for increased performance.
-
Model Safety, Evaluation, and Red Teaming: Red teaming experts assess model safety and weaknesses using task-specific metrics to gauge accuracy and identify potential improvements, then allowing for improved accuracy with new data.
-
Production User Acceptance Testing: Thorough user acceptance testing to ensure the model meets your specific requirements and delivers the expected business value.
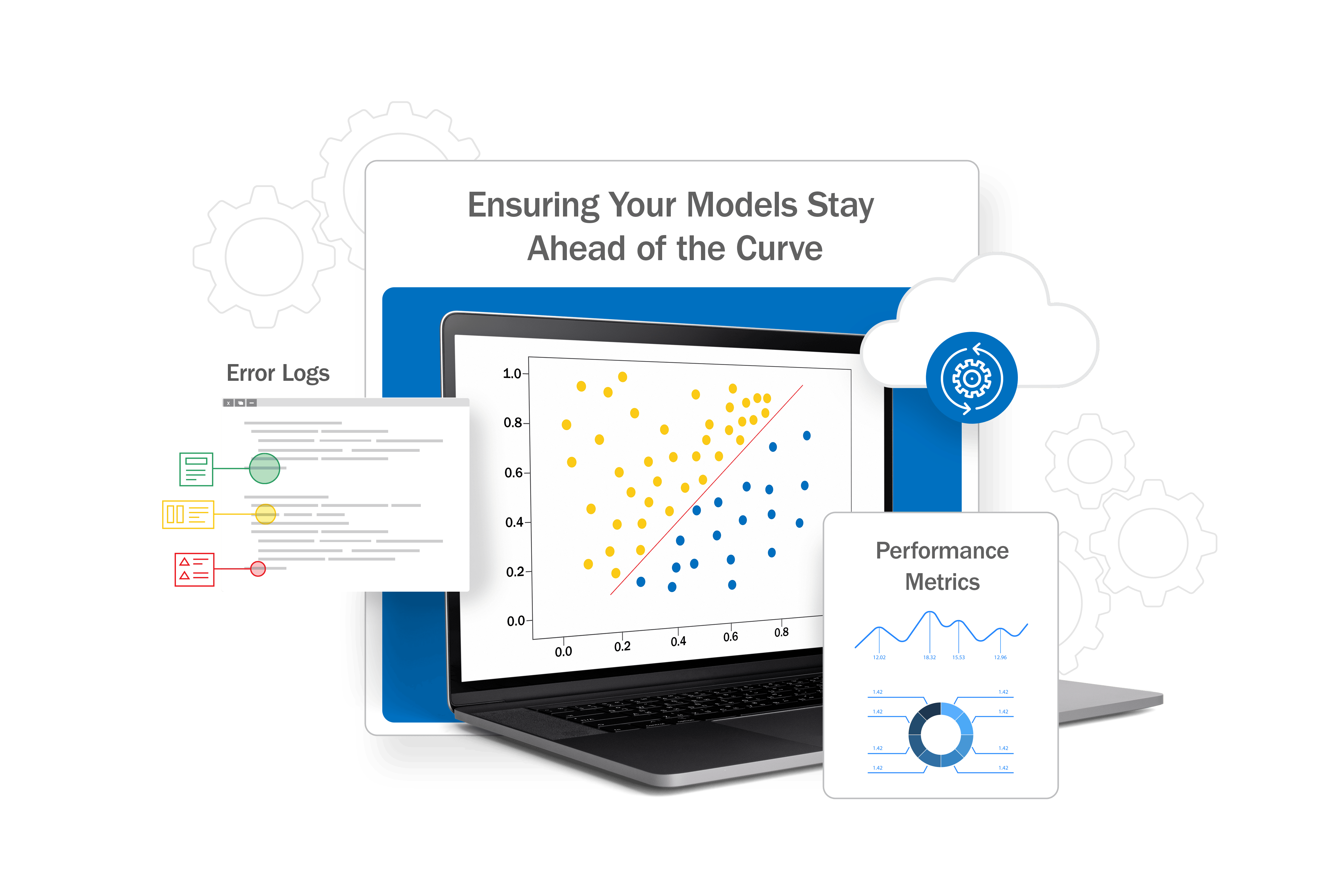
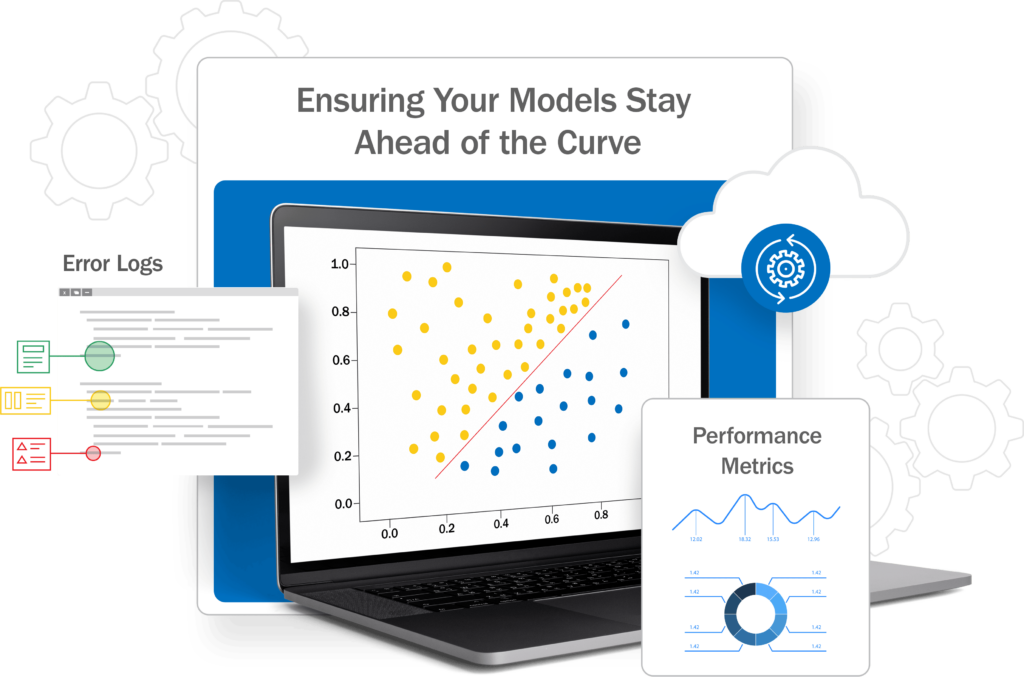
4
4 Maintain
Ensuring your models stay ahead of the curve, adapting to changing data patterns and user behaviors. Using a holistic approach, we provide ongoing maintenance into the tangible impacts of your AI initiatives. Our expertise covers critical aspects like managing model drift, model hosting, and efficient performance monitoring.
We Offer
Continuously monitoring the potential for performance degradation as data and user behavior evolve.
Regularly evaluating the model’s
performance and identifying areas for further optimization, tracking realized vs. planned benefits.
Implement robust error-handling mechanisms to ensure continued operation and address potential issues proactively.
Ensure your model is securely deployed and accessible within your infrastructure.
Streamline deployment processes for efficiency and scalability.
-
Model Drift Management: Continuously monitoring the potential for performance degradation as data and user behavior evolve.
-
Performance Monitoring: Regularly evaluating the model’s performance and identifying areas for further optimization, tracking realized vs. planned benefits.
-
Error Handling: Implement robust error handling mechanisms to ensure continued operation and address potential issues proactively.
-
Model Hosting: Ensure your model is securely deployed and accessible within your infrastructure.
-
Pipeline Automation: Streamline deployment processes for efficiency and scalability.
Empowering Businesses Across Use Cases
Innodata empowers businesses to implement generative AI models across a wide range of use cases, including:
Call Centers
Call Summarization
Customer Q&A
Sentiment Analysis
Call Analytics
Follow-Up Email Generation
Content Generation
Risk & Compliance
- Legal Document Summarization
- Case & Litigation Documents Search
- Case Documentation Relationship Analysis
- Compliance Document Search and Relationship Analysis
- Legal Assistants
- Medical Transcription and Validation
Marketing
- Content Generation
- Social Media Summarization
- Social Media Sentiment Analysis & Trends
- Campaign Content Generation
- Product Content Generation (for Sale, Documentation, Search)
HR
Sentiment Analysis
Intelligent Onboarding
Virtual Assistants
Q&A in Internal Knowledge
Management Chatbots
And More…
Deploy Leading Generative AI with Innodata’s Implementation Services
Deploy Leading Generative AI with Innodata’s Implementation Services
Case Studies