Quick Concepts: Synthetic Data
What is Synthetic Data?
Synthetic data is annotated information that is produced artificially as an alternative to real-world data. This data is created in the digital world using technology to mirror real-life sources. Though it may be artificial, synthetic data is comparable to real-world data. Research shows that it can be just as good, if not better, than data collected on actual objects, events, or people for training a machine learning (ML) model. The goal of a synthetic dataset is to be flexible and reliable enough to help train ML models.
What are the Benefits of Synthetic Data?
- Quicker processing time. Synthetic data eliminates the need to collect information from real-world occurrences, making it possible to create data and build a dataset much faster. This means that substantial amounts of data can be generated quickly.
- Automated data labeling. The time required to label data can be significantly decreased by automatically labeling the data as it is generated. Innodata’s annotation platform is built to ingest synthetic data quickly and effectively.
- Reduced costs. Businesses often face the challenge of obtaining large data sets to train an effective model in timely manner. It is expensive and time consuming to manually classify data. Synthetic data, however, can assist businesses and data scientists in overcoming these challenges and develop trustworthy ML models more quickly and at a lower cost.
- Privacy. Synthetic datasets can reduce privacy worries. With real-world data, even if sensitive or identifiable variables are removed from the dataset, other variables may still function as identifiers when they are combined, rendering data encryption ineffective. Since synthetic data is not drawn from real people or events, these identifiers do not exist, so privacy is not a problem.
- Bias prevention. Natural datasets often present biases that can spread further. Synthetic data can be added to a dataset to balance representation and mitigate bias.
- Edge cases. Synthetic data is beneficial for edge cases, which are critical to the success of a model. Edge cases are scenarios that are similar to an AI’s primary objective yet differ in significant ways. For instance, while building an image classifier, objects that are only partially visible may be regarded as edge cases.
- Modeling unforeseen events. Authentic data is always dependent on past occurrences, making it difficult to anticipate unique events in the future. Such predictions are only possible using conditional synthetic data generation, which adds conditions to the data generator creating a synthetic output that represents events that haven’t occurred in the past.
What Are the Challenges of Synthetic Data?
While using synthetic data has many benefits, there are a few challenges as well:
- Quality. While quality is always a primary concern for training data, it is especially important for synthetic data because it needs to be reliable and flexible enough to enhance model predictions. High-quality synthetic data maintains the same underlying structure and statistical distributions as the data on which it is based. Synthetic data should be indistinguishable from actual data when properly executed.
- Diverse Datasets. Variety and diversity are crucial for effective model training and simulating real-world data. For instance, in the real world an ML model may be tasked with examining invoices that could be stained, torn, or photographed in poor lighting. Synthetic invoices used for training this model should include similar features in order for the model to perform well on the ground.
- Generating Randomness. Both humans and traditional computers struggle to produce randomization well. After some time, patterns start to show up that might not accurately represent the randomness of the real world. Therefore, it will be difficult to simulate genuine randomness in synthetic data until quantum computing becomes more accessible. However, there are approaches for approximating randomness, such as conditional generative models. The key is to use the proper randomization for training.
How Do You Get Started with Utilizing Synthetic Data?
Innodata can assist you in using synthetic data for your more complex initiatives. Innodata offers end-to-end solutions for creating high-quality synthetic data across various industries. Whether you are trying to enhance your ML models or test your applications with realistic data, Innodata can provide the data you need. Visit the Innodata AI Data Marketplace to learn more about Innodata’s curated, off-the-shelf synthetic datasets.
Accelerate AI with Annotated Data
Check Out this Article on Why Your Model Performance Problems Are Likely in the Data
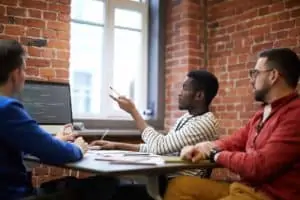
follow us