Understanding the Role of Taxonomies, Ontologies, Schemas and Knowledge Graphs
The Often-Forgotten but Critical Step in Scaling AI and Machine Learning
When most people think of artificial intelligence (AI) they conjure up notions of advanced machine learning algorithms, deep neural networks or computational cybernetics. You know, the sexy, futuristic-sounding concepts that are having an impact on the world around us. What doesn’t come to mind are taxonomies, ontologies and schemas; not as sexy, but equally if not more important in the role of bringing AI to life.
AI and machine learning require structured information to train machines to learn and understand how to replicate human behavior. The process of creating structured information from unstructured data and using it to teach machines to think like humans starts with the creation of clean structured data for AI and ML processes. Taxonomies provide the means for machines to understand hierarchies in the information. Ontologies specify the domains. Schema give clarity of how data is structured. Before we proceed, let’s break down what each term really means.
What is a Taxonomy?
A data taxonomy is the classification of data into categories and sub-categories. It provides a unified view of the data in a system and introduces common terminologies and semantics across multiple systems. Taxonomies represent the formal structure of classes or types of objects within a domain. A taxonomy is static.
What is an Ontology?
An ontology is a formal naming convention and the definition of the types, properties, and inter-relationships of the entities that really or fundamentally exist for a particular domain of discourse. An ontology is dynamic and domain-centric.
What is a Schema?
In computer programming, a schema is the organization or structure for a database. Schemas define data structure, but inference rules are separate entities within AI that define how to derive new information from existing data.
The Key Differences
The key difference between taxonomies and ontologies lies in their level of detail. Taxonomies offer a simpler classification structure, focusing on hierarchical organization. Ontologies, on the other hand, provide a richer representation of knowledge by including properties, relationships, and potentially even logical rules specific to a domain.
For example, a taxonomy for animals might classify them by kingdom, phylum, class, order, family, genus, and species. An ontology for animals, however, would not only include this classification but also define properties like “has fur,” “has wings,” or “lays eggs.” Furthermore, it could specify relationships between different animal types, such as “is a predator of” or “lives in symbiosis with.”
The Importance of Choosing the Right Tool
Selecting the appropriate tool – taxonomy or ontology – depends on the specific needs of your AI or machine learning application. Taxonomies are well-suited for tasks requiring basic organization and categorization. Ontologies are ideal for situations where deeper domain knowledge and intricate relationships between entities are crucial.
How to Get Started
The process starts with the creation of structured data from unstructured information. The first step is to acquire clean data, define a taxonomy, ontology and schema upfront. These can be done either by staring to use existing taxonomies and ontologies, or developing them from scratch. Subject knowledge and domain expertise are crucial to be able to build these correctly. This is where Innodata applies our internal subject matter expertise across domains. Once these are defined, the raw data is annotated by applying the taxonomy, ontology and/or schema as needed.
AI and ML technologies work best when the base dataset is clean, well-structured and the taxonomies and ontologies are accurate and appropriate to the context. Subject matter expertise and domain knowledge are key ingredients for success. Unfortunately, finding the right combination of all the above in one place is quite a task in itself. Open-source taxonomies and ontologies could be too generic and might not be the best choice. In-house SMEs could be working on day-to day-functions and not available for crucial projects. With the high velocity and variety of data flowing in, taxonomies need to be updated and renewed constantly for organizations to remain relevant and sustain continued AI performance. Innodata has a full spectrum of solutions to help build clean data , create base taxonomies from scratch, SMEs across a large variety of domains for ontologies and schema development, cutting edge-tools to build and update taxonomies, ontologies, annotate raw data, customize, test and validate in an agile process, which could drastically accelerate the time to market by 40-50%.
At the end of the day, machines cannot read, interpret, or make sense of data without structure. A well-designed taxonomy, ontology and schema are fundamental to teach machines to understand patterns like humans and are fundamental for long-term AI and ML success.
Start a live chat with an expert.
Bring Intelligence to Your Enterprise Processes with Generative AI
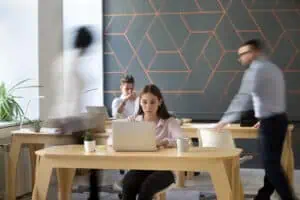
Whether you have existing generative AI models or want to integrate them into your operations, we offer a comprehensive suite of services to unlock their full potential.
follow us