Quick Concepts
What is Explainable AI?
Artificial intelligence (AI) has become the engine driving innovation across industries, but its opaque nature can leave businesses feeling like passengers in a self-driving car. Explainable AI (XAI) emerges to shed light on the inner workings of these algorithmic oracles.
Traditionally, AI models have functioned as black boxes, churning out predictions with nary a whisper of “why.” This lack of transparency hinders trust, leading to concerns about bias, fairness, and ultimately, the reliability of AI-driven decisions. XAI steps in, bridging the communication gap between algorithm and human.
Why Does Explainable AI Matter?
Trust is the linchpin of any successful business relationship, and XAI fosters it by empowering businesses to explain the decisions made by AI. Imagine telling a customer why their loan was turned down because of a certain data point used by the AI. XAI makes these outcomes clear, helping to build trust and strong relationships. It shows what factors the AI used to make its predictions, which helps in fixing, fine-tuning, and improving the AI’s performance.
Unveiling Bias and Ensuring Fairness
AI algorithms, like any human-crafted tool, are susceptible to biases embedded in the data they train on. XAI shines a spotlight on these potential discrepancies, enabling businesses to identify and mitigate them. This proactive approach ensures unbiased decision-making, protects against legal and ethical risks, and builds a reputation for responsible AI implementation.
Optimizing Performance
XAI isn’t just about holding AI accountable; it’s about making it a more insightful and effective tool. By understanding the factors influencing predictions, businesses can identify weaknesses and fine-tune models for improved performance. It could pinpoint specific data points that negatively impact customer churn predictions and use XAI to refine the model for higher accuracy. This iterative process leads to continuously evolving AI that delivers maximum business value.
How Does Explainable AI Work?
There’s no single XAI method, different techniques cater to various models and purposes. Let’s explore some popular approaches:
- Model-specific Methods: These techniques are tailored to the specific architecture of a particular AI model. For example, analyzing the weights and connections in a neural network can reveal which features heavily influence its predictions.
- Model-agnostic Methods: These versatile tools can be applied to any AI model, regardless of its internal workings. Techniques like LIME (Local Interpretable Model-Agnostic Explanations) or SHAP (SHapley Additive exPlanations) provide localized explanations for individual predictions, highlighting the most important features contributing to the outcome.
- Counterfactual Explanations: “What if my credit score was different?” XAI can show what would happen in these ‘what-if’ situations. It provides different results and helps us see how changes in inputs affect what we get out. This helps us understand how the AI thinks and find any biases it might have.
- Attention Mechanisms: Some AI models, like language processing specialists, track their “focus” as they analyze data. XAI taps into these mechanisms, revealing which parts of the input data the model deems most crucial for its predictions.
Use Cases
Applications of XAI are diverse, here are just a few areas where it shines:
- Financial Services: Imagine XAI as a financial advisor explaining a complex credit score. It guides fairer lending decisions, identifies potential bias, and safeguards against risk. With XAI, financial institutions build deeper client trust and navigate the increasingly complex financial landscape with confidence.
- Healthcare: Demystify AI-powered medical diagnoses and empower both doctors and patients to engage in informed conversations, understand treatment plans, and build trust in AI-driven healthcare solutions. This shines a light into the black box of medical algorithms, leading to personalized care and improved patient outcomes.
- Marketing: Ever wondered why some ads resonate while others fall flat? XAI holds the answer. It analyzes how specific customer profiles respond to targeted campaigns, helping marketers optimize budgets and personalize experiences for higher engagement and conversion rates. Think of it as a marketing microscope, revealing the hidden patterns that drive customer behavior.
- Cybersecurity: XAI can shed light on how AI detects anomalies and threats, enabling IT teams to improve security measures and respond to incidents more effectively.
Embracing the Future
In a world increasingly powered by AI, understanding how it works matters. Explainable AI builds trust, ensures fairness, and unlocks AI’s true potential.
Unleash Explainable AI with Innodata:
- Build trusted models: Our expert team creates interpretable AI solutions, helping you understand outputs and build user trust.
- Ethical data foundation: Leverage diverse, ethically sourced datasets to combat bias and ensure responsible AI.
- Optimize for success: Gain deep insights into AI performance with XAI techniques, continuously refining your results.
Contact us today to achieve transparent AI success!
Bring Intelligence to Your Enterprise Processes with Generative AI
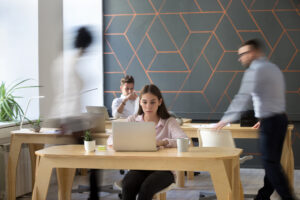
Whether you have existing generative AI models or want to integrate them into your operations, we offer a comprehensive suite of services to unlock their full potential.
follow us