An Introduction to 3D Lidar
Humans have developed a variety of technologies to observe and analyze our surroundings. These technologies come with different capabilities, limitations, and use cases. One such tech is lidar (light detection and ranging), which is based on laser light reflection. Although lidar has been available for over 50 years, its full potential and applications are still being discovered. Recently, Apple added a lidar sensor to the cameras on its iPhone 12 Pro and iPad Pro, bringing lidar technology into the hands of everyday users. While lidar still faces challenges in autonomous vehicle navigation, this technology has a stunning array of use cases and is a powerful adjunct to other imaging and object detection methods.
Lidar Basics
Lidar uses laser light to measure distances. A transmitter emits pulses of light, which reflect off surfaces and return to a receiver. The receiver records the time taken for each pulse of light to return, which is used to calculate the distance traveled by each beam. These times and distances yield a set of data points called a point cloud. A point cloud can then be converted into a 3D image that depicts surfaces, landforms, structures, and objects. Lidar can detect a variety of materials, including hard objects as well as chemical compounds and clouds. Lidar scanners can be airborne or terrestrial, topographic (examining dry terrain using a near-infrared laser), or bathymetric (mapping underwater terrain using green water-penetrating light). They can be mobile (mounted on a moving object such as a vehicle or drone) or static (mounted on a stationary surface). Lidar mapping is extremely accurate, depicting surfaces and objects with 2-3cm accuracy even in long-range applications.
Lidar Capabilities and Applications
Because object detection and ranging are fundamental to so many applications, the variety of use cases for lidar is overwhelming. To date, lidar has well-known applications in aviation, geophysical mapping, and autonomous vehicle navigation. Here is a partial list of its additional uses:
- Ecology/Conservation – forest management, wildfire analysis and management, pollution studies
- Alternative Energy – planning and optimization of solar energy systems based on location and vegetation analysis; increasing wind farm output by measuring wind speeds
- Agriculture – crop mapping, seed and fertilizer optimization, insect monitoring, plant species classification (for weed control)
- Meteorology – assessment of clouds, winds, and atmospheric chemicals and gases
- Law Enforcement – speed tracking; crime scene scanning to show objects, blood, and bullet trajectories
- Disaster Management – assessment and management of floods, earthquakes, wildfires, mudslides, etc.
- Infrastructure and Transportation – building and assessing bridges and roads, developing traffic networks
- Archeology – mapping archeological sites, revealing unseen structures and artifacts
- Robotics – perception of the environment, navigation, object classification
- Oil and Gas Exploration – finding oil and gas deposits by examining chemicals in the atmosphere
- Video Gaming/Virtual Reality/Augmented Reality – enhancing simulations of landscapes and scenes with highly accurate lidar data
- 3D Photography – creating 3D photos of objects and scenes for use in measurement, 3D modeling, interior/layout design, construction/remodeling, art, and recreational photography.
Advantages of Lidar
Lidar is a mainstay of aviation navigation and geophysical mapping, and its primary benefits are most apparent in long-range mapping applications:
Accuracy
Lidar scans can produce immensely detailed and accurate measurements even at great distances; current terrestrial scanners claim to achieve 5mm accuracy at a distance of 2500m.
Speed
Compared to manual human measurement and mapping, lidar scanning generates a wealth of data at lightning speed. This is especially significant in surveying and mapping applications (imagine human surveyors collecting forestry data, climbing individual trees to measure their height), as well as industrial scanning and modeling.
Safety
Safety increases exponentially when humans no longer need to be physically present on-site to measure landforms and water bodies, assess the layout of industrial plants, or analyze electrical lines, roadways, or traffic conditions. The more these processes can be automated and remotely controlled, the safer they become.
Cost
Lidar applications are far more cost-effective than human labor for large-scale surveying and mapping, since the slow work of massive crews of humans can be achieved quickly by a single lidar scanner. In small-scale use cases, lidar currently suffers from high equipment costs, but this will change as lidar technology and hardware evolve. For example, it would be impractical to purchase specialized lidar scanning equipment for a home remodeling or construction job, but lidar 3D modeling is already becoming more accessible for small-scale projects now that lidar is available on iPhones and iPads.
Challenges of Lidar
Lidar has a proven track record for producing highly accurate 3D representations of landscapes and objects. However, 3D lidar data collection still presents some challenges for autonomous vehicle navigation. These include:
Inclement Weather
Lidar sensors are sensitive to rain, snow, and fog and may produce incomplete or inaccurate data in these conditions. Even in normal weather conditions, early morning fog and brightly lit objects against white backgrounds cause problems for lidar sensors and receivers. For this reason, autonomous vehicles currently combine multiple types of sensors (such as lidar, radar, and sonar) rather than relying on a single ranging technology.
Surface Reflectivity
Within a given range, the accuracy of lidar sensor data (number of laser pulses returned to the receiver) varies based on the reflectivity of various surfaces. Textured, or “diffuse” surfaces, such as asphalt and clothing, produce predictable reflections, and hence work well for lidar. “Retro-reflective” surfaces, including paint markings on roads, reflective road signs, and animals’ eyes, are also good targets for lidar because the light beams return without much signal loss. However, “specular” surfaces like mirrors, glass, and smooth water produce very small, poorly dispersed reflections that often miss the receiver, causing inaccuracies in lidar measurements.
Vibration
Platform movement and vibration also influence some lidar scanner measurements. On mobile forms of lidar – like autonomous vehicles – sensors must maintain performance through vibrations and shocks as well as normal wear and cleaning. While vibration absorbers and sensors can mitigate and compensate for the effects of mechanical vibrations, bumps, and sudden movements, uneven terrain continues to present a challenge for terrestrial lidar applications.
Lidar Annotation
Lidar relies on ML (machine learning) models to provide real-time interpretations of point cloud data. Analyzing 3D lidar point cloud data is a complex, repetitive, and time-consuming process. In addition, point clouds are often sparse and low resolution, making object identification ambiguous. For this reason, lidar applications are only practical and valuable when ML models do the heavy data processing. Training these ML models, however, requires large volumes of accurately annotated training data, manually labeled by human annotators. 3D Lidar point cloud annotation requires more complex analysis and labeling than 2D image and video annotation, including the following:
- Lidar semantic segmentation, where each point in a point cloud must be identified and classified according to the object to which it belongs.
- Lidar box labeling, where cuboid boxes are created to denote vehicles and other objects for autonomous vehicles, allowing machine learning algorithms to easily identify these objects in new datasets. Cuboid annotation can be applied to 2D camera and video footage to indicate the depth of objects, essentially adding 3D information to a 2D image for ML training.
- Lidar landmark annotation, which labels anatomical or structural features of objects, allowing ML algorithms to determine the shape of objects of varying sizes, including smaller ones.
- Lidar polygon annotation, which creates polygons that are precisely drawn around objects of interest, such as signs, logos, and humans in different postures, to train object detection and localization algorithms.
- Lidar polyline annotation, which labels road markings to help autonomous vehicles detect traffic lanes on highways and city streets.
Lidar Fusion
While lidar provides critical depth and distance measurements on its own, it is even more powerful when combined with other imaging modalities such as videography, photography, radar, and sonar.
3D Lidar and 2D Image/Video Fusion
Lidar’s distance measurements provide accurate 3D representations but lack visual detail and richness; conversely, 2D photography and videography provide rich visual information but lack accurate depth and distance information. This is why some geophysical mapping devices, such as the DJI ZenMuse and the Phantom 4 RTK, fuse lidar and photogrammetry (photography for surveying and mapping) in UAVs (Unmanned Aerial Vehicles, or drones) to produce accurate topographical images for professional land surveys of vegetated areas. In addition, personal devices now combine lidar and traditional photography to allow everyday smartphone and tablet users to produce unique 3D photos.
Lidar Plus Radar and Sonar
Lidar scanning also works well in conjunction with radar and sonar. The combined modalities allow for increased sensory range and enhanced performance in poor weather conditions, in water, and when tracking fast-moving objects. Consequently, autonomous vehicles such as those used by Waymo and Uber use a combination of these scanning methods (in addition to GPS trackers) to enable autonomous vehicle navigation and obstacle avoidance.
Conclusion
Lidar is a versatile, established technology with a wide range of practical applications. It recently made its way into the hands of smartphone users via the iPhone 12 and 13 pro and the iPad Pro, increasing public awareness of its capabilities. Lidar is already automating and revolutionizing fields such as geophysical mapping and environmental management, but some technical challenges remain before its widespread use can be safe for autonomous driving and cost-effective for small-scale applications. In addition, lidar data annotation is a complex, detailed, and labor-intensive process. High-quality data annotation for lidar ML model training will become increasingly important as lidar expands its footprint in industry, business, and personal use. Overall, lidar technology is on a rapid growth trajectory and just beginning to hit its stride. As hardware, deployment methods, and machine learning models improve, lidar use will drive significant advancements across industries, making it a smart investment for the not-so-distant future.
Accelerate AI with Annotated Data
Check Out this Article on Why Your Model Performance Problems Are Likely in the Data
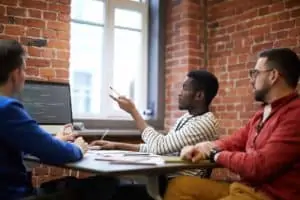
follow us