Quick Concepts: Explainable AI
What is Explainable AI?
Explainable AI (XAI) refers to machine learning models in which decision-making processes are transparent and understandable; where model outputs, whether accurate or faulty, can be broken down and explained. These models are sometimes referred to as “white box models.” In contrast, “black box models,” such as neural networks, run enormous amounts of data through deep, convoluted pathways that can be virtually impossible to understand or trace. They often produce decisions that even designers cannot explain. Explainability is becoming an increasingly important component of ethical AI as artificial intelligence systems are tasked with critical decision-making in a variety of industries. In medical diagnostics and autonomous vehicle navigation, for example, AI predictions could have life and death consequences.
What are the Benefits of Explainable AI?
Transparency and explainability in AI models can yield multiple benefits, including:
- Improving overall model performance by making errors, biases, and drift easier to trace and correct
- Enhancing a model’s credibility and users’ trust in its predictions, because users can understand how decisions and predictions are made
- Enabling a model to fulfill ethical, legal, or organizational requirements
- Providing data insights by allowing more visibility into the drivers of AI outcomes, such as patterns in user behavior
- Making it possible to question, examine, and challenge or change AI outcomes in situations where users are adversely affected by AI decisions
What are Some Strategies for Making AI Explainable?
Based on research by the US Defense Advanced Research Project Agency (DARPA), explainable AI is divided into three components:
- Prediction accuracy – where models produce accurate results and behave as expected
- Traceability – visibility into how the model has arrived at its decisions
- Decision understanding – ensuring that users understand how the model makes decisions
The goals and standards of explainable AI vary based on the use case; more critical use cases should be held to a higher explainability standard. Some basic guidelines that may help move businesses toward explainable AI include:
- Thoughtful data selection and curation to minimize errors and bias
- Use of the simplest, most transparent AI model for a given task (i.e., matching the technology to the application)
- Ensuring that a model operates only under the conditions for which it was designed
- As far as possible, striving for a model that can provide “accurate,” “meaningful,” and “understandable” explanations for its decisions and outputs (based on guidelines from the National Institute of Standards and Technology)
- Ensuring that the above information is accessible to users
Explainable AI is an evolving concept that has emerged due to the breakneck development of AI technology. This rate of advancement can cause fallout in the form of errors, biases, confusion, and distrust. Explainable AI seeks to bridge the gap between AI models and users so that AI predictions feel less like opaque machine outputs and more like extensions of human decisions.
Accelerate AI with Annotated Data
Check Out this Article on Why Your Model Performance Problems Are Likely in the Data
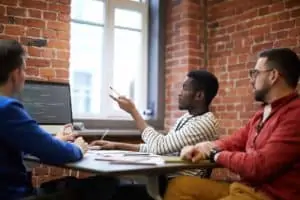
follow us