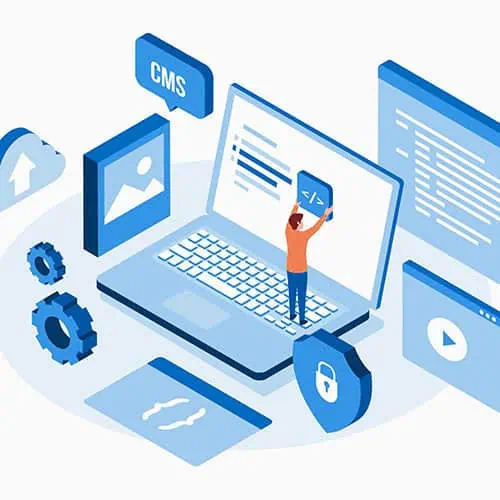
Whitepaper & Insights
4 Steps to Build Truly Intelligent Machine Learning Models
Explore the 4 different types of data annotation modalities being used to create training data for machine learning.
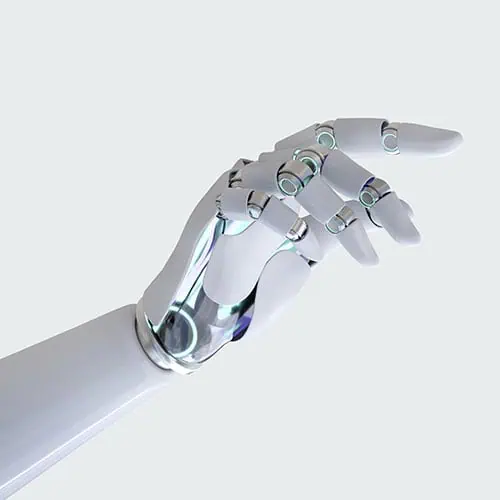
Whitepaper & Insights
4 Critical Requirements for Building Truly Intelligent AI Models
Think you're ready for AI? Make sure you have these 4 critical steps in order.
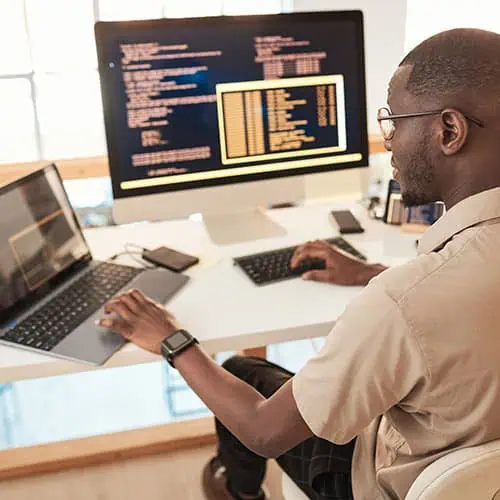
Whitepaper & Insights
5 Questions to Ask Before Getting Started with Data Annotation
To Read Full Whitepaper, Submit Your Information Below.
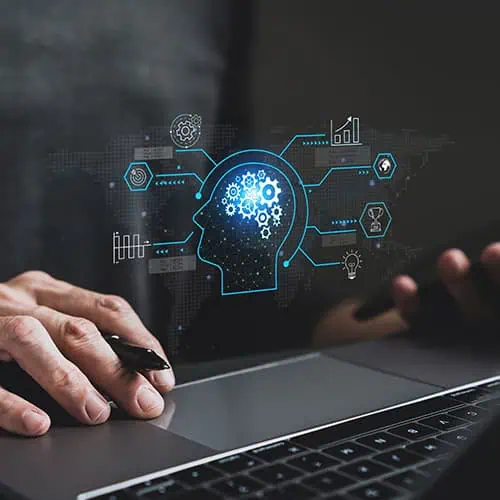
Whitepaper & Insights
4 Steps to Build Truly Intelligent Machine Learning Models
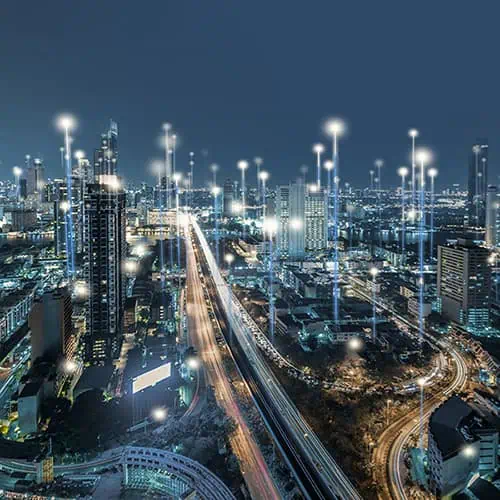
Whitepaper & Insights
Accelerate AI with Data Annotation
Whitepaper & Insights
The Art & Science of Data Annotation
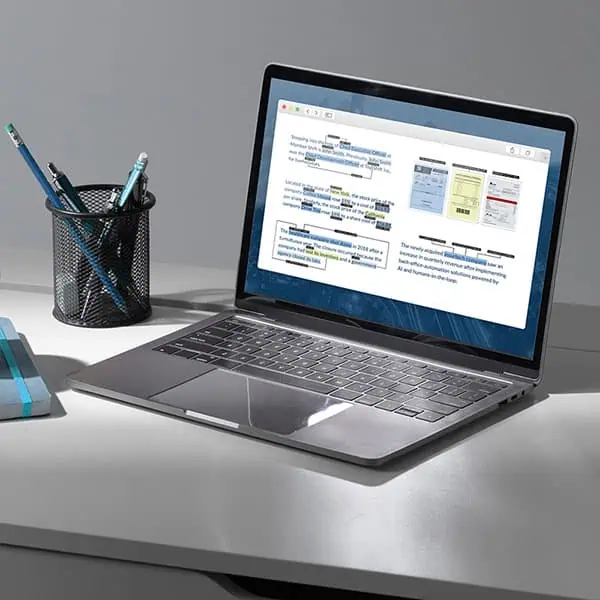
Whitepaper & Insights
Zoning Documents for Data Extraction
To Read Full Whitepaper, Submit Your Information Below.
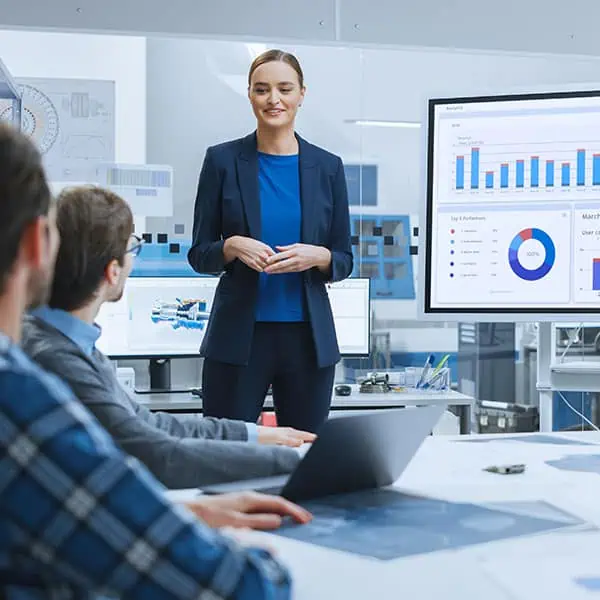
Whitepaper & Insights
Data Challenges in AI: User Perspectives and Insights
To Read Full Whitepaper, Submit Your Information Below.
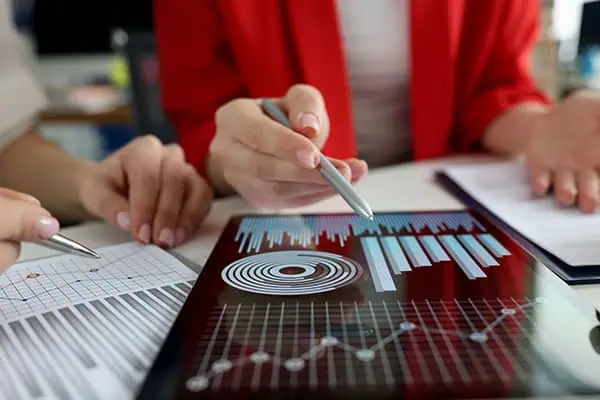
To Read Full Whitepaper, Submit Your Information Below.
Whitepaper & Insights
The Latest Framework for Building Datasets & Driving AI Accuracy
The Principles of a Data-Centric Approach to AI
There is no shortage of hype around AI — and with good reason. AI aims to redefine how information is collected, integrated, and analyzed; ultimately leading to more informed insights and delivering better outcomes. But for all its hype, integrating AI remains a challenge for many companies. While the media focuses on models and algorithms, the data aspect is not as prominently discussed. According to a recent paper by Google researchers, “data is the most under-valued and de-glamorized aspect of AI.”
If you are reading this whitepaper, you clearly understand the importance of data — it is what makes artificial intelligence truly intelligent. The challenge is getting the right data to continuously train your machine learning models. Successful AI projects demand an accurate foundation on which to build and train machine learning algorithms. In this paper, we will reveal the best framework to build a sustainable, quality data pipeline to ensure the success of your AI projects. Then we will examine the newest data-centric strategy and why it is essential for building a high performing AI model. Finally, you will find a curated list of resources to source relevant data for your unique use cases.
Resources
Whitepapers & Insights
Insights and best practices for unraveling the complexities of digital data.
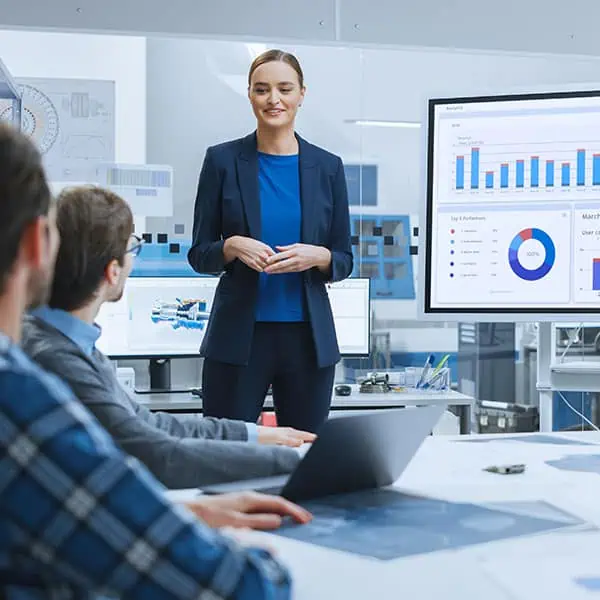
User Interviews
Data Challenges in AI: User Perspectives and Insights
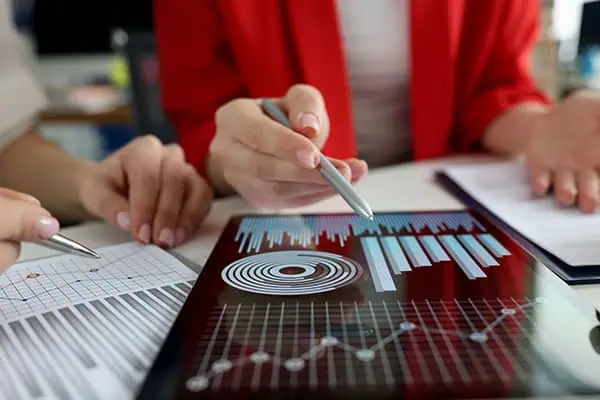
Whitepaper
The Latest Framework for Building Datasets & Driving AI Accuracy
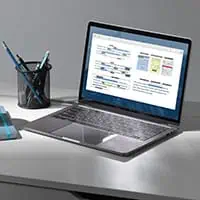
Whitepaper
Zoning Documents for Data Extraction
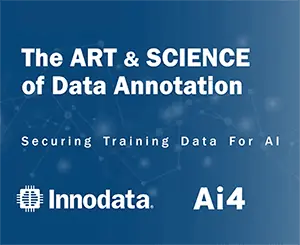
Webinar
The Art & Science of Data Annotation
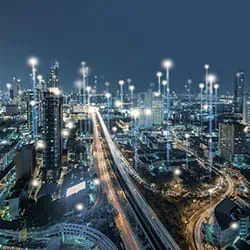
Ebook
Accelerate AI with Data Annotation
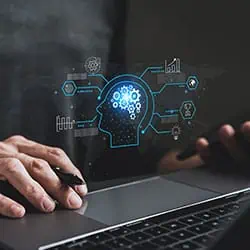
Ebook
Four Steps to Build Truly Intelligent Machine Learning Models
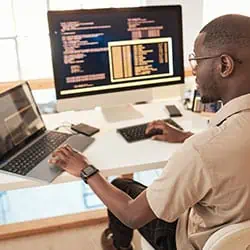
Slideshare
Five Questions to Ask Before Getting Started with Data Annotation
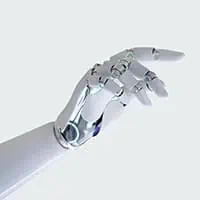
Slideshare
Four Critical Requirements for Building Truly Intelligent AI Models
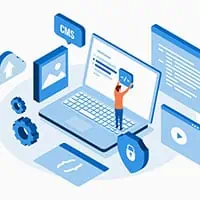