How Do You Source Training Data for Generative AI?
At the heart of any successful generative AI model lies high-quality training data. Sourcing the right training data is an important step in the development of powerful AI models. In this article, we’ll explore the intricacies of sourcing training data for generative AI, why it matters, and how Innodata can help you navigate this essential aspect of AI development.
The Role of Training Data in Generative AI
Before delving into the sourcing process, let’s understand the vital role of training data in generative AI models. Generative AI models learn to generate human-like text by analyzing vast amounts of text data during training. They derive patterns, grammar, context, and semantics from this data, enabling them to generate coherent and contextually relevant text.
The quality, diversity, and quantity of training data directly impact the performance of a generative AI model. High-quality data helps the model generate more accurate and coherent text, while a diverse dataset allows it to handle a broader range of topics and styles. Lastly, an ample amount of training data contributes to the model’s overall proficiency.
How to Source Training Data
Let’s explore how to source training data effectively for your generative AI projects, considering the specific tasks and use cases:
Determine Specific Tasks:
Before sourcing training data, it’s essential to determine the specific tasks that your model aims to perform. The type of training data you source should align with these tasks. For instance, if your project involves tasks like summarization or question answering, you’ll need a dataset that reflects these. This could mean sourcing datasets that contain long-form content for summarization tasks, or datasets with question-answer pairs for question-answering tasks.
Define Use Cases:
The use cases for a generative AI model also dictate the types of data to be sourced. For example, if you’re developing an LLM for customer support chatbots, you would require conversational datasets. These datasets, which contain real-world examples of customer support interactions, can help train your model to understand and generate appropriate responses in a customer support context.
On the other hand, if your AI model is intended for image captioning, you would need a dataset consisting of image and caption pairs. This type of dataset can help your model learn to associate specific images with appropriate descriptive text, enabling it to generate accurate and relevant captions for new images.
Now, let’s delve into how to source training data effectively for your generative AI projects:
- Curated Datasets: One of the most efficient ways to source training data is through curated datasets. These datasets are carefully selected, organized, and cleaned to ensure high quality and relevance to your project. Models trained on diverse, high-quality data tend to perform better and generate more meaningful results. Organizations like Innodata specialize in creating and curating datasets tailored to specific AI applications.
- Web Scraping: Web scraping involves extracting data from websites and online sources. It can be a valuable method for sourcing training data, especially for text-based generative AI projects. However, it’s essential to respect ethical guidelines and copyrights when scraping data from the internet.
- Data Annotation Services: Data annotation involves labeling or tagging data to make it suitable for AI training. This process can be time-consuming and requires expertise. Outsourcing data annotation to professionals can save you time and ensure the data is labeled accurately.
- In-House Data Collection: In some cases, you may need to collect data in-house, especially if your project requires domain-specific or proprietary information. This approach allows you to have full control over the data collection process but can be resource intensive.
- Data Augmentation: Data augmentation involves expanding your training dataset by creating variations of existing data. This technique can be useful when working with limited data but requires careful implementation to maintain data quality.
- Data Privacy and Compliance: Prioritize data privacy and compliance with relevant regulations. This is particularly important when working with user-generated or sensitive data. For example, if you are a financial institution, you must ensure that the data used to train a generative AI model complies with financial data protection regulations.
- Outsourcing Data: Working with trusted partners, such as Innodata, provides access to otherwise inaccessible data sources.
How is Reward Modeling Used?
Reward modeling is utilized in numerous areas of generative AI. Let’s look at a few examples:
Natural Language Processing: Reward modeling helps AI models produce more coherent and contextually relevant content. This is especially important in applications like chatbots, content generation, and language translation.
Content Creation: Reward modeling can be applied to creative content generation, such as music composition or graphic design, ensuring that AI-generated art aligns with artistic standards and user preferences.
Drug Discovery: In pharmaceutical research, generative AI models can use reward modeling to generate chemical structures for potential new drugs. The reward signal can be based on predicted drug efficacy and safety.
Dialogue Systems: Reward modeling can help improve the performance of AI dialogue systems or chatbots by rewarding responses that are relevant, informative, and engaging.
Types of Training Data for Generative AI
Sourcing training data for generative AI often involves selecting the appropriate type of data for your specific use case. Here are some common types of training data:
Text Data: Text data is essential for models like GPT, which generate written content. Sources for text data can include books, articles, websites, social media, and more. These corpora should cover various topics, styles, and languages to ensure a broad understanding of human language. For a business, text data can be sourced from customer interactions, product descriptions, and industry-specific documents. For example, a content generation platform might source text data from a wide range of web articles and blogs to train a model for generating blog posts and articles automatically.
Domain-Specific Data: In many cases, it’s important to use domain-specific data to train generative AI models. For applications in specialized fields like healthcare, finance, or law, it’s crucial to source data specific to that domain. This ensures the AI model can generate contextually accurate text. For example, a medical research institution might source medical journals and research papers to train a generative AI model for automatically summarizing complex medical texts.
User-Generated Content: Social media posts, user reviews, and forum discussions are rich sources of data for training generative AI models. They capture informal language and various perspectives, making the model more versatile.
Multimodal Data: In addition to text, you can enhance your AI model’s capabilities by incorporating images, audio, and video data. Sourcing such data requires combining various data sources. This is especially useful for tasks like image captioning or generating multimedia content. For example, a social media platform might use a combination of user-generated text and images to train an AI model that generates image captions based on textual input.
Structured Data: Data in structured formats, such as databases or spreadsheets, can be converted into text data for training. This is useful for AI applications that require generating reports or summaries from structured information.
Image Data: Sourcing diverse image data is vital for generative AI models like DALL-E, which are designed to produce images from text descriptions. This can come from publicly available images, datasets, stock photos, and in-house collections. An e-commerce company might use image data from its product catalog, stock photos, and user-generated content to train an AI model that generates product images based on textual descriptions.
Challenges of Sourcing Training Data and Best Practices
Sourcing training data for generative AI models presents several challenges, but there are best practices to overcome these.
Challenges include ensuring high-quality and accurate data, as low-quality or erroneous data can lead to biased or nonsensical output from the AI model. Strict adherence to data privacy regulations like GDPR is necessary when dealing with sensitive or personal information. It’s crucial to anonymize and protect user data. The diversity of the data is also an important aspect to consider for the versatility of the AI model, but sourcing diverse data can be challenging, especially in niche domains. Generative AI models require massive amounts of training data, which can be resource-intensive to acquire and manage. Lastly, it’s important to ensure that you have the necessary rights and licenses to use the data for training purposes, especially when using copyrighted material.
To overcome these challenges, consider the following best practices:
Diversify Your Sources: Ensure that your training data comes from a wide range of sources, including public datasets, proprietary data, and crowdsourced content. Diverse data sources help the model generalize better.
User Consent and Bias Mitigation: If you plan to use user-generated content, ensure you have proper consent and anonymize the data to protect user privacy. Be vigilant about bias mitigation to ensure the data used for training is representative and unbiased.
Collaborations: Collaborate with organizations, institutions, or researchers who may have access to domain-specific data that you need. Collaborations can help pool resources and data, enabling a more comprehensive dataset for your generative AI model.
Data Preprocessing: Invest time and effort in data preprocessing to ensure data quality. This step may involve removing duplicates, correcting errors, and standardizing formats. Consider using language translation services for text data preprocessing, aligning sentence structures, correcting spelling errors, and converting text to a common format.
Data Cleaning and Labeling: Invest time in cleaning and labeling your training data to remove noise and ensure accuracy.
Data Generation: Consider using generative AI to create synthetic data when real-world data is scarce or limited. This can help supplement your training datasets and ensure you have sufficient data for effective model training.
Continuous Learning: Sourcing training data is not a one-time task. To keep your generative AI model up-to-date and competitive, you must continuously update your training data. Language evolves, new topics emerge, and user preferences change. By regularly refreshing your dataset, you ensure that your AI model remains relevant and effective.
Outsourcing vs. Internal Sourcing
When it comes to sourcing training data for generative AI, organizations are faced with an important decision: internal sourcing or outsourcing.
Internal sourcing offers control but demands resources and expertise in data collection, annotation, preprocessing, and compliance with data privacy regulations.
On the other hand, outsourcing to a specialized vendor like Innodata can be a strategic choice. Innodata’s teams have extensive experience in sourcing and handling training data for AI projects. We ensure high-quality and diverse datasets, adhere to data privacy regulations, and can scale our services as your project evolves. Outsourcing to Innodata allows your team to focus on model development and innovation.
As a leader in data management and AI, Innodata provides comprehensive solutions for sourcing training data for generative AI projects. Offering curated datasets, data annotation services, and prioritizing ethical data sourcing. By partnering with Innodata, you can develop generative AI models that deliver exceptional results while upholding ethical standards and data privacy.
Ready to take your generative AI projects to the next level? Leverage Innodata’s expertise in sourcing training data and focus on what you do best – innovating. Don’t miss out, contact us today and lay the foundation for AI solutions that truly make a difference.
Bring Intelligence to Your Enterprise Processes with Generative AI
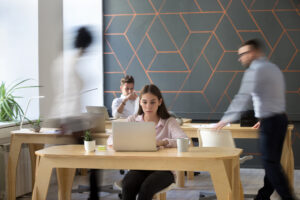
Whether you have existing generative AI models or want to integrate them into your operations, we offer a comprehensive suite of services to unlock their full potential.
follow us